일 | 월 | 화 | 수 | 목 | 금 | 토 |
---|---|---|---|---|---|---|
1 | 2 | 3 | 4 | 5 | ||
6 | 7 | 8 | 9 | 10 | 11 | 12 |
13 | 14 | 15 | 16 | 17 | 18 | 19 |
20 | 21 | 22 | 23 | 24 | 25 | 26 |
27 | 28 | 29 | 30 |
- 디퓨전모델
- RNN
- WinAPI
- Diffusion
- 모션매칭
- dl
- motion matching
- deep learning
- Font Generation
- animation retargeting
- Unreal Engine
- NLP
- ue5.4
- Stat110
- CNN
- 딥러닝
- ddpm
- BERT
- 오블완
- 생성모델
- 언리얼엔진
- userwidget
- 폰트생성
- multimodal
- Few-shot generation
- Generative Model
- cv
- GAN
- UE5
- WBP
- Today
- Total
Deeper Learning
Attention Mechanism 본문
Attention
seq2seq 모델에서 RNN, LSTM, GRU 모두 초기시점의 정보가 희석되고 Decoder로 전달되는 Encoder의 마지막 hidden state (+ cell state) 하나에 모든 Encoder의 input의 정보를 담기가 어려워 정보 손실이 일어난다.
이를 해결하기 위해 seq2seq 모델에서 Decoder에서 output 각각의 계산이 모두 Encoder의 hidden state를 참고하여 이루어지는 Attention Mechanism이 제시되었다.
기계번역의 예시에서 Encoder가 특정 단어를 input으로 받아 도출한 output은 상응하는 단어를 번역하는 Decoder가 예측을 하는데 필요한 input과 유사할 것이라는 가정하에 만들어진 Attention Mechanism은 기존 seq2seq 모델보다 좋은 성능을 보였다.
Attention Model
Attention 모델은 Encoder의 어느 input에 집중하는지를 나타내는 Attention score를 구하는데 이를 구하는 방식에 따라 여러가지 Attention 모델로 나뉜다.
(h = Encoder의 hidden state, s = Decoder의 hidden state)
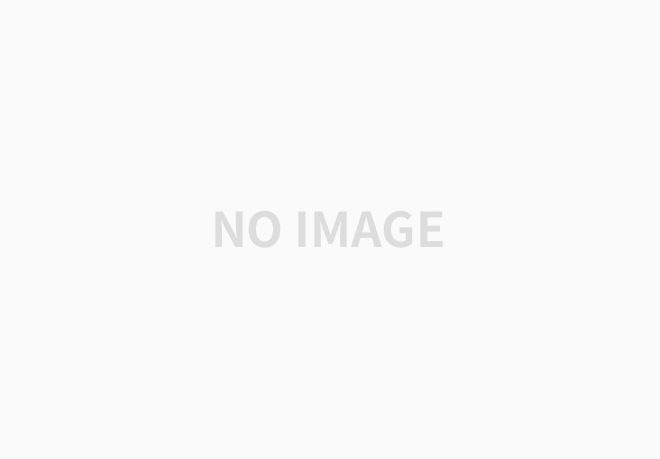
Attention model의 구조는 Attention score를 구하는 방법에 따라 양방향 순환 신경망을 사용하거나, Concatenate 또는 Dense net을 통과시키는 등 다양하다.
아래의 모델은 양방향 순환 신경망을 사용하여 Encoder의 양방향의 hidden state를 concatenate 시킨 vector를 hi로 한다.
Encoder의 모든 시점의 hidden state H와 Decoder의 전 시점의 hidden state인 st−1를 Attention layer에 input으로 하여 얻은 context vector를 Decoder의 input으로 활용한다.
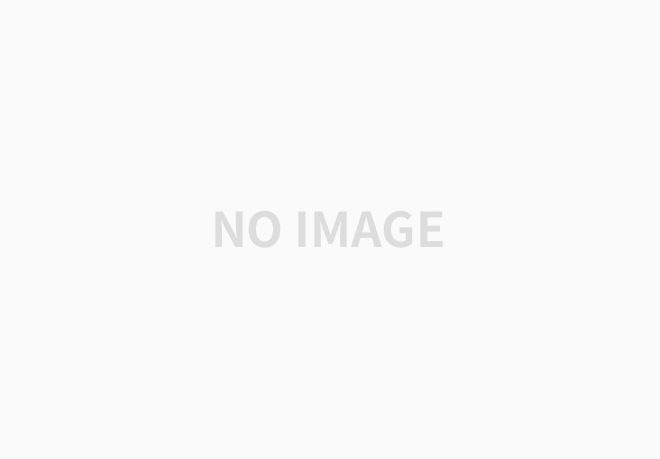
Attention score α의 계산은 st−1과 ai를 concatenate 한 vector를 Dense layer에 input으로 하고 Softmax 함수를 통해 이를 전체 합이 1이 되는 확률의 값으로 변환한다. 이렇게 구해진 Attention score는 현재 Decoder의 시점의 단어를 예측하는데 Encoder의 어느 token에 "집중"하는지 나타낸다.
a는 양방향 순환 신경망의 hidden state를 concatenate 한 vector로 이를 decoder의 hidden state와 concatenate하기 위해서는 Decoder의 hidden unit의 차원이 Encoder의 hidden unit의 차원의 2배가 되어야 한다. (현재 설명하는 특정 Attention model에만 해당되는 내용)
이렇게 구한 Attention score를 해당하는 Encoder의 hidden state인 a에 곱하고 이를 모두 더해 context vector를 만들고 이를 Decoder의 input으로 사용한다.
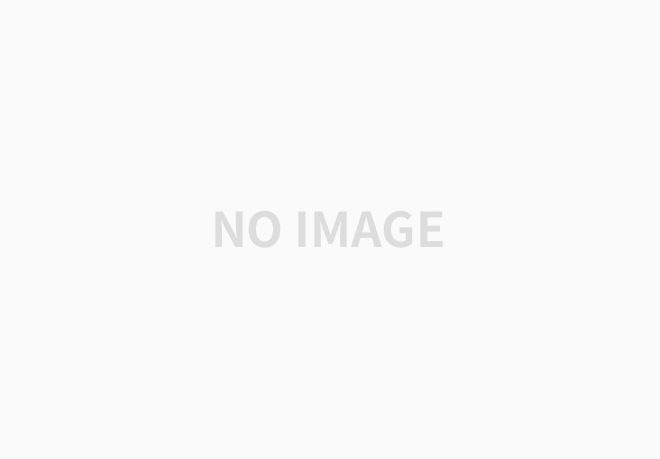
Attention Score를 시각화하여 나타낸 Attention map은 특정 단어를 예측할 때 Encoder의 어느 단어에 집중하였는지 나타낸다.
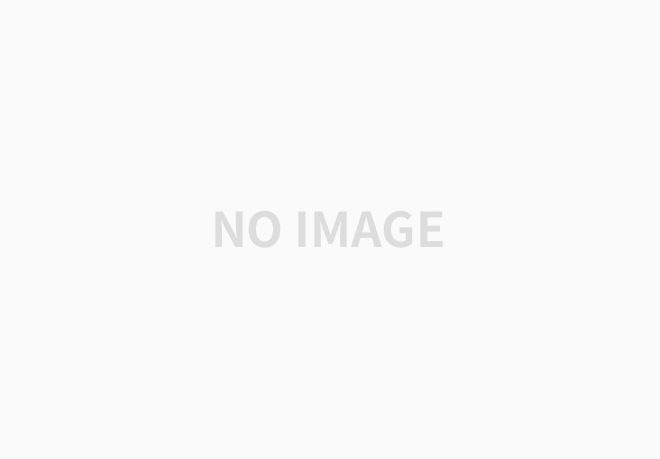
YYYY-MM-DD 형태로 자연어를 변환하는 학습된 모델의 Attention map에서 -05를 예측하는데 input의 M에 집중한 것을 볼 수 있다.
Tensorflow Implements
약 3천개의 질문과 답을 기록한 간단한 데이터셋을 사용하였다.
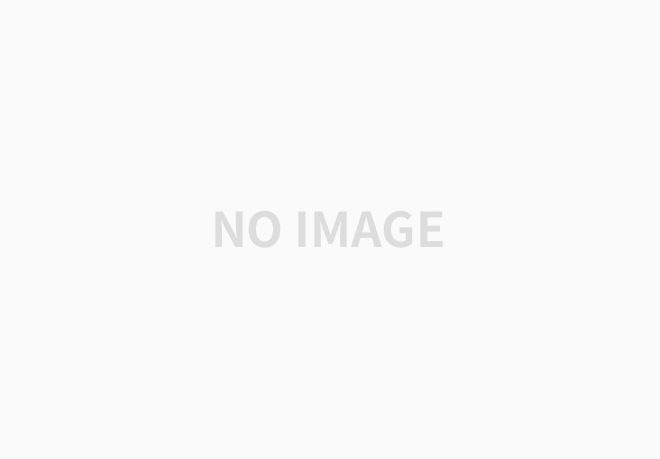
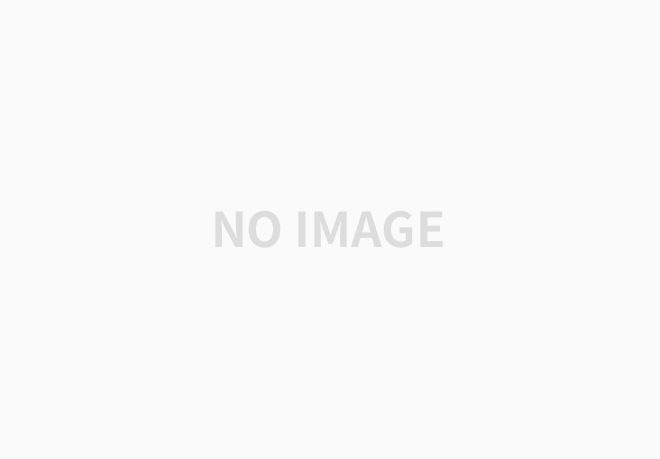
Sequence의 길이를 12로 고정하고 0으로 padding 하였으며 decoder target의 경우 문장의 마지막에 End token인 2를 추가하였다.
class Encoder(tf.keras.layers.Layer):
def __init__(self, vocab_size, embedding_dim, enc_units, batch_size):
super(Encoder, self).__init__()
self.batch_size = batch_size
self.vocab_size = vocab_size
self.embedding_dim = embedding_dim
self.enc_units = enc_units
self.concat = tf.keras.layers.Concatenate(axis=-1)
self.embedding = tf.keras.layers.Embedding(self.vocab_size, self.embedding_dim)
self.pre_bi_lstm = tf.keras.layers.Bidirectional(tf.keras.layers.LSTM(self.enc_units,return_sequences=True, return_state=True))
def call(self, x):
# x shape == (batch_size, seq len)
# h,c = hidden
# h c shape == (batch_size, enc_units)
x = self.embedding(x)
# embedded x shape == (batch_size, seq_len, emb_dim)
x,fh,fs,bh,bs = self.pre_bi_lstm(x)
# x shape == (batch_size, seq_len, enc_units*2)
# fh,fs,bh,bs shape == (batch_size, enc_units)
return x
class Attention(tf.keras.layers.Layer):
def __init__(self,seq_len):
super(Attention, self).__init__()
# self.units = units
self.seq_len = seq_len
self.repeat = tf.keras.layers.RepeatVector(self.seq_len)
self.concat = tf.keras.layers.Concatenate(axis=-1)
self.d1 = tf.keras.layers.Dense(10,activation='tanh')
self.d2 = tf.keras.layers.Dense(1,activation='relu')
self.softmax = tf.keras.layers.Softmax(axis=1)
def call(self, a, s_prev):
# a.shape == (batch_size, seq_len, enc_units*2)
# s_prev.shape == (batch_size, dec_units)
s_prev = self.repeat(s_prev)
# s_prev.shape == (batch_size, seq_len, dec_unit)
a_s = self.concat([a,s_prev])
# a_s.shape == (batch_size, seq_len, dec_units*2)
out = self.d1(a_s)
# out.shape == (batch_size, seq_len, dense units)
out = self.d2(out)
# out.shape == (batch_size, seq_len, dense units==1)
attention_score = self.softmax(out)
# attention_score.shape == out.shape
context_vector = attention_score * a
# context_vector.shape == (batch_size, seq_len, dec_units)
context_vector = tf.reduce_sum(context_vector, axis=1)
# context_vector.shape == (batch_size, dec_units) == decoder input
context_vector=tf.expand_dims(context_vector,1)
return context_vector, attention_score
class Decoder(tf.keras.layers.Layer):
def __init__(self,vocab_size, dec_units, batch_size):
super(Decoder, self).__init__()
# self.batch_size = batch_size
self.vocab_size = vocab_size
self.dec_units = dec_units
self.lstm = tf.keras.layers.LSTM(self.dec_units,return_state=True)
self.d1 = tf.keras.layers.Dense(self.vocab_size,activation='softmax')
def call(self,x,hidden):
h,c = hidden
# h,c shape == (batch_size, dec_units)
# x.shape == (batch_size, 1, dec_units)
output, new_h, new_c = self.lstm(x,initial_state=[h,c])
# 3 output shape == (batch_size, dec_units)
output = self.d1(output)
# output shape == (batch_size, vocab_size)
return output, new_h, new_c
class AttentionMachineTranslationModel(tf.keras.Model):
def __init__(self,vocab_size, embedding_dim, enc_units, dec_units, batch_size, seq_len,end_token=2):
super(AttentionMachineTranslationModel, self).__init__()
self.end_token=end_token
self.encoder = Encoder(vocab_size,embedding_dim,enc_units,batch_size)
self.attention = Attention(seq_len)
self.decoder = Decoder(vocab_size,dec_units,batch_size)
self.enc_units = enc_units
self.dec_units = dec_units
def call(self, x):
a = self.encoder(x)
predict = []
h = tf.zeros((x.shape[0],self.dec_units))
c = tf.zeros((x.shape[0],self.dec_units))
for t in range(x.shape[1]):
context, attention = self.attention(a,h)
output, h, c = self.decoder(context, [h,c])
predict.append(output)
return tf.stack(predict,axis=1)
def predict(self, x):
a = self.encoder(x)
predict = []
attentions = []
h = tf.zeros((x.shape[0],self.dec_units))
c = tf.zeros((x.shape[0],self.dec_units))
for t in range(x.shape[1]):
context, attention = self.attention(a,h)
output, h, c = self.decoder(context, [h,c])
predict.append(output)
attentions.append(attention)
return [tf.stack(predict,axis=1),attentions]
이해를 돕기위해 layer마다 shape를 기록하였다.
tf.keras의 순환 신경망 layer의 return_sequences는 모든 시점에서 hidden_state를 반환하며, return_state는 마지막 hidden state (cell state)를 반환한다.
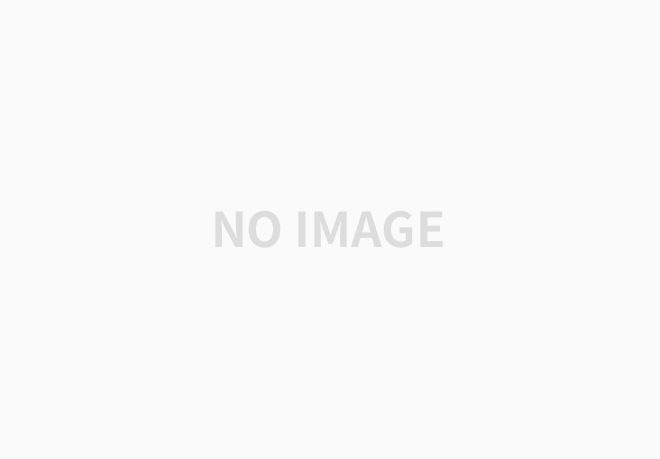
학습 시간과 GPU 자원의 부족으로 3천개의 희소한 데이터로 훈련 시킨 결과 Overfitting이 발생하였지만 Attention 알고리즘의 구현을 확인 할 수 있었다.
Reference
[1] Cho et al. (2016). Neural Machine Translation by jointly Learning to Align and Translate. arXiv:1409.0473v7
[2] Andrea Galassi et al. (2019). Attention in Natural Language Processing.
[3] 어텐션 메커니즘, ratsgo.github.io/from%20frequency%20to%20semantics/2017/10/06/attention/
'AI > Deep Learning' 카테고리의 다른 글
Autoencoder (0) | 2021.02.11 |
---|---|
Gradient Descent (0) | 2021.02.10 |
Text similarity Model (CNN, MaLSTM) (0) | 2020.12.30 |
Deep Convolutional Generative Adversarial Networks (DCGAN) (0) | 2020.12.28 |
BLEU (Bilingual Evaluation Understudy Score) (0) | 2020.12.24 |