일 | 월 | 화 | 수 | 목 | 금 | 토 |
---|---|---|---|---|---|---|
1 | 2 | 3 | 4 | 5 | ||
6 | 7 | 8 | 9 | 10 | 11 | 12 |
13 | 14 | 15 | 16 | 17 | 18 | 19 |
20 | 21 | 22 | 23 | 24 | 25 | 26 |
27 | 28 | 29 | 30 |
- CNN
- 오블완
- Font Generation
- Stat110
- 폰트생성
- BERT
- ue5.4
- 언리얼엔진
- cv
- 모션매칭
- 생성모델
- animation retargeting
- dl
- Generative Model
- Few-shot generation
- RNN
- deep learning
- 디퓨전모델
- WinAPI
- multimodal
- Unreal Engine
- 딥러닝
- Diffusion
- GAN
- userwidget
- motion matching
- NLP
- WBP
- ddpm
- UE5
- Today
- Total
목록Font Generation (7)
Deeper Learning
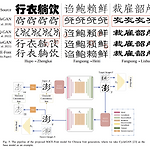
Jie Zhou, Yefei Wang, Yiyang Yuan, Qing Huang, Jinshan Zeng (2022.11) Abstract 효과적인 가이드 정보가 없어 GAN-based 모델이 mode collapse 문제를 겪고 있음 본 연구는 skeleton guided channel expansion(이하 SGCE) 모듈로 skeleton 정보를 generator에게 channel expansion 방식으로 주어 local, global structure를 파악하게 하는 새로운 guidance 방식을 제시 SGCE 모듈을 통해 mode collapse 문제가 완화되었으며 실험을 통해 이를 보임 4개의 metrics에서 SOTA를 달성 다른 중국어 폰트 생성 모델에도 plug-and-play 모듈로..
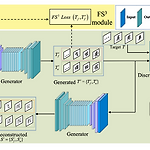
Jinshan Zeng, Yefei Wang, Qi Chen, Yunxin Liu, Mingwen Wang, and Yuan Yao, (2022.11) Abstract 현재 중국어 폰트 생성의 주류 방식은 GAN 기반 모델, 하지만 GAN 기반 모델은 mode collapse 문제를 겪고 있음 이 문제를 해결하기 위해 one-bit stroke encoding과 few-shot semi-supervised scheme으로 local, global structure 정보를 찾아내는 방식을 제시 해당 아이디어를 기반으로 본 논문은 stroke encoding과 few-shot semi-supervised scheme을 CycleGAN과 결합하여 mode collapse 문제를 완화한 StrokeGAN+를 제..
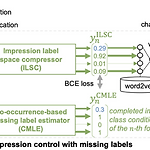
Seiya Matsuda, Akisato Kimura, Seiichi Uchida, Kyushu Univ., NTT, (2022.03) Abstract impression labeling 된 dataset을 사용하여 specific impression fonts를 GAN으로 생성하는 것이 목표 Main difficulty font impression은 애매 특정 impression label이 없다고 그 폰트가 해당 impression에 해당하지 않는 것이 아님 (dataset이 불안정) Key Idea co-occurrence-based missing label estimate impression label space compressor MyFonts 데이터셋은 전문가 + 비전문가가 tagging 한 ..
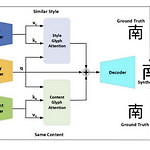
Under review as a conference paper at ICLR 2022 Mingtao Guo, Wei Xiong, Zheng Wang, Yong Tang, Ting Wu. Xian Univ. (2021.09) Abstract Non-local neural network에서 영감을 받아 제시한 GANet content encoder와 style encoder는 content glyph과 style glyph에서 key와 value를 추출 기존 SOTA few-shot font 생성 모델을 뛰어넘는 결과 Introduction 중국 폰트에 대한 인기가 세계적으로 증가하면서 니즈또한 증가하고 있음 그러나 폰트 생성은 노동집약적이며 오랜시간이 걸림 image to image translation과..
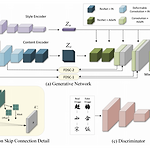
Abstract 현존 methods는 대부분 supervised learning, 매우 많은 paired data가 필요 image-to-image translation은 style을 텍스쳐와 색깔로 정의 냉림 Feature Deformation Skip Connection (FDSC)를 제시 predict pairs of displacement map employs the predicted maps to apply deformable convolution to the low-level feature maps from content encoder style-invariant feature 표현을 학습하기 위해 content encoder에 3개의 deformable convolutional layers를..
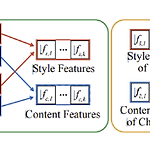
ABSTRACT few-shot generation(FFG)는 2가지 조건을 만족하여야 한다. target char의 global structure 보존 diverse local reference style의 표현 explicit 하게 component label을 주지 않고 MX-Font는 multiple style features를 추출한다. multiple expert들이 different local concepts을 표현한다. 각 expert들이 different local style에 특화될 수 있도록 weak supervision방식으로 component label을 이용한다. 각 expert들에게 component assign problem을 graph matching problem으로 정의..
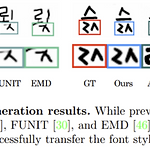
ABSTRACT 글자가 많은 언어에 대해 새 폰트를 만드는 것은 매우 노동집약적이고 시간이 많이 든다. 현존하는 폰트 생성 모델은 많은 reference image를 참고하지 못하면 디테일한 스타일을 살리지 못한다. 조합형 글자에 대해 적은 수의 샘플을 보고 하이퀄리티의 글자를 생성해내는 DM-Font를 제시하겠다 better quality, faithful stylization compared to SOTA font generation methods Introduction GAN base image-to-image translation 방식으로 폰트를 생성하는데 전의 method는 775개의 samples이 필요했다 기존 방식은 pre-trained된 모델에 새로운 reference image를 사용하..