일 | 월 | 화 | 수 | 목 | 금 | 토 |
---|---|---|---|---|---|---|
1 | 2 | 3 | 4 | 5 | ||
6 | 7 | 8 | 9 | 10 | 11 | 12 |
13 | 14 | 15 | 16 | 17 | 18 | 19 |
20 | 21 | 22 | 23 | 24 | 25 | 26 |
27 | 28 | 29 | 30 |
Tags
- animation retargeting
- Generative Model
- RNN
- ddpm
- 언리얼엔진
- ue5.4
- 생성모델
- WBP
- userwidget
- 디퓨전모델
- 오블완
- Unreal Engine
- 모션매칭
- GAN
- deep learning
- Font Generation
- Diffusion
- cv
- WinAPI
- Stat110
- NLP
- UE5
- 폰트생성
- 딥러닝
- Few-shot generation
- BERT
- CNN
- multimodal
- dl
- motion matching
Archives
- Today
- Total
목록diffusion 수식정리 (1)
Deeper Learning
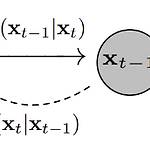
prerequisite: Diffusion Model에 대한 기초적인 이해 Diffusion Model T = 전체 Timesteps 수 xT = forward process를 T 번 적용한 마지막 Timestep T 에서의 이미지 x0 = 원본 이미지 Forward Process q(xt|xt−1)=N(xt,√1−βtxt−1,βtI) β 는 noise(variance)의 강도를 조절하는 parameter로 DDPM 논문에서는 0.0001 ~ 0.02의 값을 사용 β 가 선형적으로 timesteps에 따라(DDPM에서는 linear noise scheduler 사용) 0.0001에서 0.02까지 증가하..
AI/Deep Learning
2023. 4. 8. 12:30