일 | 월 | 화 | 수 | 목 | 금 | 토 |
---|---|---|---|---|---|---|
1 | 2 | 3 | 4 | 5 | ||
6 | 7 | 8 | 9 | 10 | 11 | 12 |
13 | 14 | 15 | 16 | 17 | 18 | 19 |
20 | 21 | 22 | 23 | 24 | 25 | 26 |
27 | 28 | 29 | 30 |
- animation retargeting
- cv
- 모션매칭
- Unreal Engine
- motion matching
- UE5
- multimodal
- Stat110
- Generative Model
- 오블완
- Font Generation
- WBP
- userwidget
- CNN
- 디퓨전모델
- ue5.4
- 생성모델
- GAN
- RNN
- 딥러닝
- 언리얼엔진
- NLP
- BERT
- dl
- deep learning
- 폰트생성
- Diffusion
- WinAPI
- Few-shot generation
- ddpm
- Today
- Total
Deeper Learning
ELMo (Embeddings from Language Model) 본문
Beyond Embeddings
- Word2Vec, Glove 임베딩은 13~14년에 인기
- 많은 task에서 좋은 성능을 보였음
- Problem: representations are shallow
- 첫 번째 layer만 all Wikipedia data로 pretrained한 embedding의 이점을 가짐
- 나머지 layer (LSTMs)는 pretrained data를 보지 못하고 own data로만 학습됨
- Bank Account와 River Bank에서 Bank는 전혀 다른 의미를 가지지만 Word2Vec 또는 Glove로는 이를 반영하지 못하고 Bank가 같은 벡터로 임베딩 되어 사용됨
- 문맥을 반영한 워드 임베딩 (Contextualized Word Embedding)이 필요하다.
→ embedding layer만 pre-train 하지않고 더 많은 layer를 pretrain하여 words를 더 명확하게 학습. ( "to rule" vs "a rule"에서 rule을 구분), 문법도 학습 (형용사의 위치, 부사의 위치 등).
Deep contextualized word representations
ELMO (Embeddings from Language Model)
ELMo는 2018년에 제안된 "워드 임베딩 방법론"
Pre-trained language model을 사용하여 Contextualized word embedding
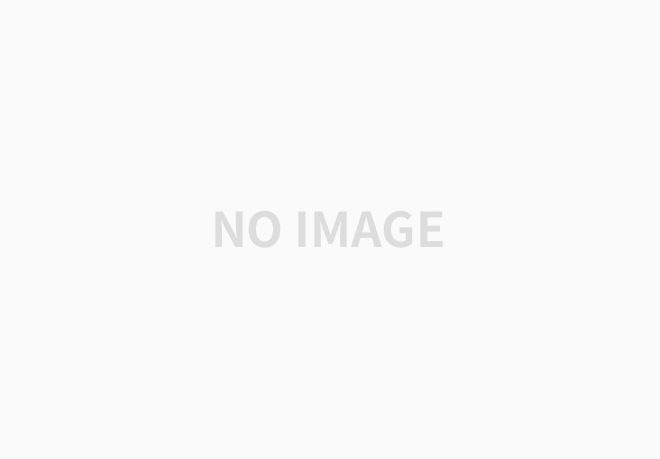
순방향, 역방향 multi-layer LSTM을 모두 사용하는 biLM (Bidirectional Language Model)을 사용.
하지만 Bidirectional LSTM 처럼 순방향, 역방향 hidden state를 concat 시키는 구조가 아닌 2개의 순방향, 역방향 언어 모델을 학습시키는 구조.
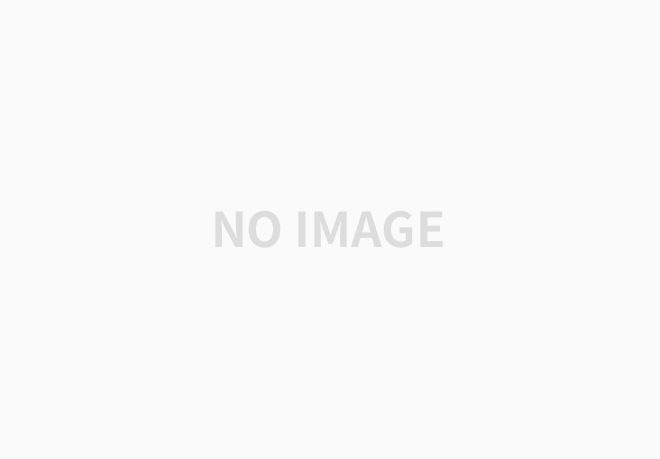
biLM의 input을 위한 word embedding은 charCNN이라는 방법을 사용
CharCNN
character 단위로 계산하여 마치 subword를 참고하는 것처럼 문맥에 상관없이 run, running의 연관성을 찾아낼 수 있는 임베딩 방법론.
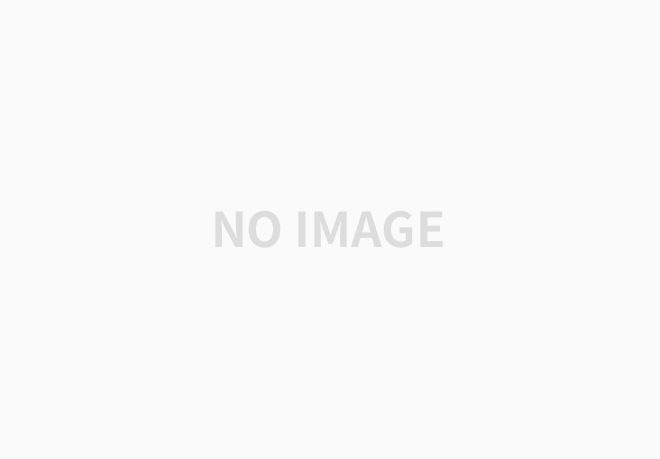
좌측 사진처럼 Character Lookup Table을 만든다.
행의 차원은 262 (알파벳과 special token, 특수문자 등을 모두 포함), 열의 차원은 임베딩 차원인 16으로 설정되어있다.
우측 사진처럼 word "cat"을 벡터로 변환하려면 <BOW> c a t <EOW> <PAD> <PAD> ... 처럼 시작과 끝을 알리는 special token과 padding token을 추가해준다.
패딩으로 인해 차원은 동일하게 (262 x 16)이 된다.
그 후 CNN을 사용하여 Feature map을 생성한다.
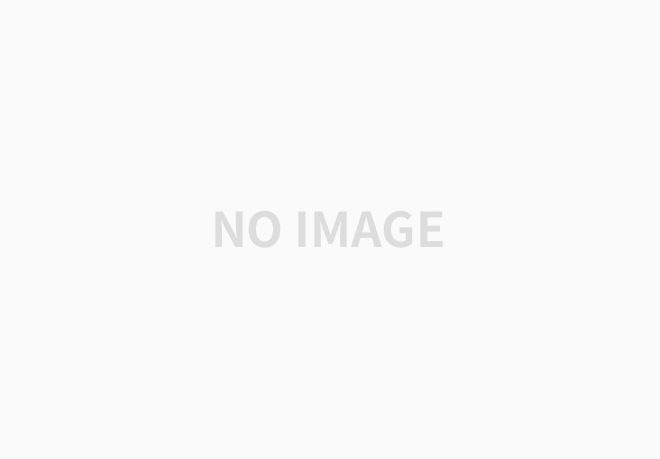
[region size, num of filters]가 [1, 32], [2, 32], [3, 64], [4, 128], [5, 256], [6, 512], [7, 1024]로 설정되어있다면 마지막에 1-max pooling 후 concat한 결과의 shape은 ( 1, 2048 )이 된다. (32 +32 +64+128+256+512+1024 = 2048)
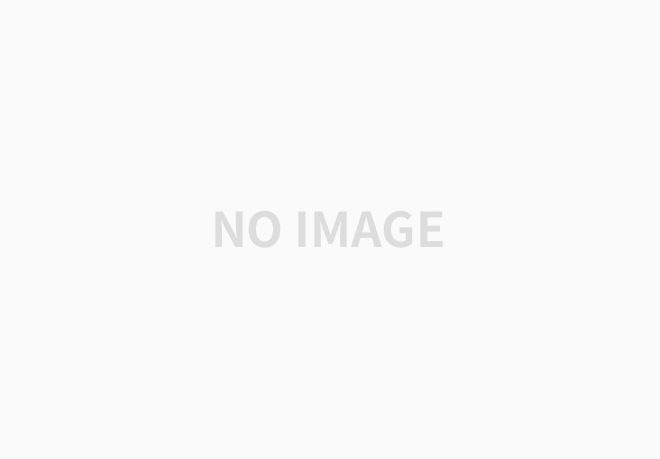
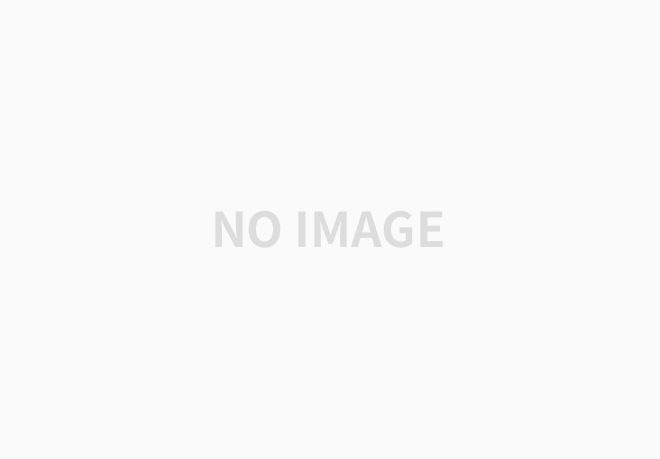
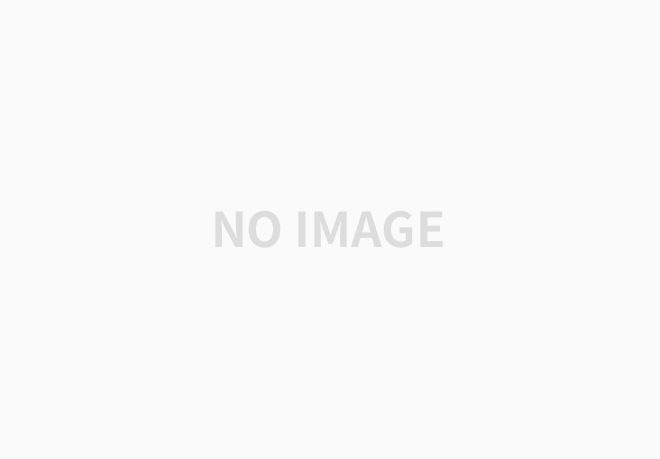
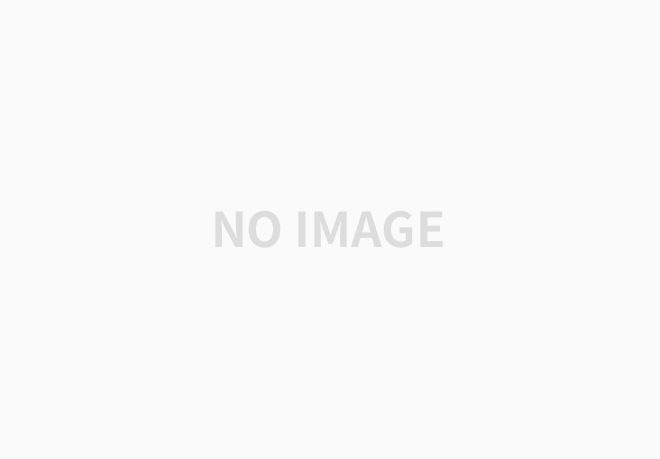
play의 ELMo representation이 완성되었다. 가중치인 s와 scalar term 감마는 학습되는 parameter이며 ELMo representation을 만들 때 pre-trained LM은 freeze 시킨다.
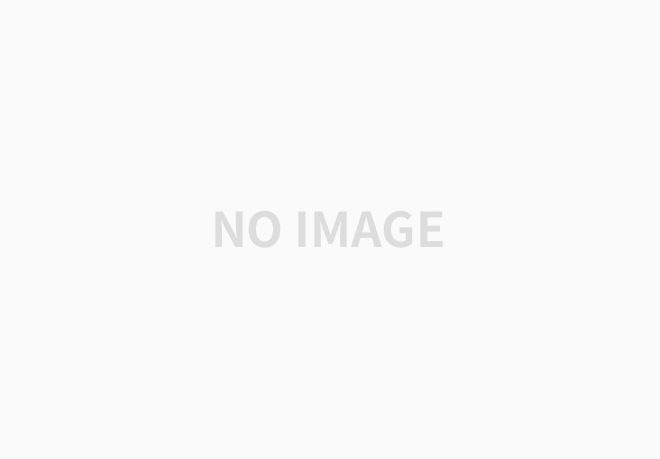
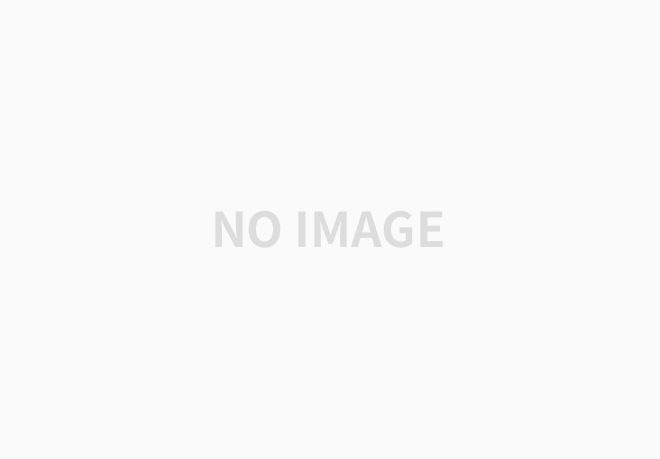
기존에 임베딩 레이어 한 층만 pre-trained 시키는 method의 문제를 해결, high-quality word representation을 얻기 위한 임베딩 방법론으로, pre-trained biLM의 다층 모델 구조 전체를 임베딩을 위해 사용한다.
semantic feature를 더 잘 capture 할 수 있는 장점이 있으며 실제로 여러 task에 대한 성능도 크게 향상되었음.
Reference
[1] Full Stack Deep Learning Lecture 4
[2] 딥러닝을 이용한 자연어 처리 입문 https://wikidocs.net/33930
[3] Mattew E. Peters et al. (2018). Deep contextualized word representations, https://arxiv.org/pdf/1802.05365.pdf
'AI > Deep Learning' 카테고리의 다른 글
Squeeze-and-Excitation Networks (SE-Net) (0) | 2021.10.14 |
---|---|
Attention is all you need: Transformer (0) | 2021.10.10 |
Full Stack Deep Learning - Lecture 1 ~ 3 (0) | 2021.10.07 |
Universal Approximation Theorem (0) | 2021.10.02 |
DG-Font: Deformable Generative Networks for Unsupervised Font Generation (0) | 2021.09.25 |