일 | 월 | 화 | 수 | 목 | 금 | 토 |
---|---|---|---|---|---|---|
1 | 2 | 3 | 4 | 5 | ||
6 | 7 | 8 | 9 | 10 | 11 | 12 |
13 | 14 | 15 | 16 | 17 | 18 | 19 |
20 | 21 | 22 | 23 | 24 | 25 | 26 |
27 | 28 | 29 | 30 |
Tags
- motion matching
- multimodal
- 디퓨전모델
- 딥러닝
- animation retargeting
- 폰트생성
- Unreal Engine
- RNN
- 오블완
- userwidget
- 모션매칭
- CNN
- ue5.4
- Stat110
- GAN
- Font Generation
- dl
- cv
- Generative Model
- ddpm
- deep learning
- Few-shot generation
- NLP
- BERT
- WinAPI
- Diffusion
- 언리얼엔진
- 생성모델
- WBP
- UE5
Archives
- Today
- Total
Deeper Learning
ArcFace: Additive Angular Margin Loss for Deep Face Recognition 본문
AI/Deep Learning
ArcFace: Additive Angular Margin Loss for Deep Face Recognition
Dlaiml 2021. 12. 13. 08:262018, Stefanos Zafeiriou Imperial College London
Jiankang Deng et al.
Abstract
- Deep Convolutional Neural Networks를 사용하여 large-scale face recognition에서 feature learning을 할 때 challenge는 discriminative power를 향상시키기 위한 loss function 설계
- Centre loss는 deep features와 해당 class의 centre 사이의 Euclidean space에서 distance에 penalty를 주어 intra-class compactness를 달성
- Sphere Face는 last fc layer의 linear transformation matrix가 angular space에서 class centres의 representation이 된다는 가정하에 deep features와 해당 weight의 angle에 penalize
- face class를 분리하기 위해 잘 설계된 loss function과 함께 margin을 사용하는 것이 최근 연구의 방향
- 얼굴인식을 위한 highly discriminative features를 얻을 수 있는 Additive Angular Margin Loss (ArcFace)를 제시
- ArcFace는 hypersphere에서 geodesic distance(지오데식 거리, 그래프의 두 꼭짓점의 최단 경로)와 정확히 일치하기 때문에 기하학적 해석이 가능
- 여러 Face dataset에서 SOTA 달성
1. Introduction
- 얼굴 인식을 위한 DCNN은 크게 두 방향이 있음
- softmax를 사용하는 multi-class classifier
- triplet loss 등을 사용하여 embedding을 바로 학습
- large-scale train data에서 softmax-loss-based methods와 triplet-loss-based methods는 모두 좋은 성능을 냄
- 그러나 각각 단점이 있음
- Softmax loss는 linear transformation matrix의 차원이 인식해야하는 사람 수에 따라 선형적으로 증가
- 학습된 deep features가 closed-set에서 잘 나뉘어있지만 open-set에서 성능은 알 수 없음
- Triplet loss는 large-scale datasets에서 조합의 수가 매우 많아지며 효과적인 학습을 위한 semi-hard sampling 방법을 찾는 것이 매우 어렵다
- softmax loss의 discriminative power를 향상시키기 위한 연구가 많았음
- 각 feature vector와 해당 class의 median과 유클라디안 거리를 사용하여 intra-class compactness를 얻고 softmax loss에서 joint penalisation을 사용하여 inter-class dispersion을 달성
- face classes가 데이터가 많아짐에 따라 증가하여 하지만 학습 도중 centre를 업데이트하는 것이 매우 어려움
- Sphereface, CosFace도 안정적인 학습, 적용하기 용이, 해석 가능 측면에서 완벽하지 않았음
- ArcFace
- Engaging: normalised hyperspace에서 angle과 arc의 distance, geodesic distance를 직접적으로 optimize
- Effective: SOTA 벤치마킹 스코어
- Easy: 적은 양의 코드로 구현이 가능. 어떤 data에도 쉽게 수렴
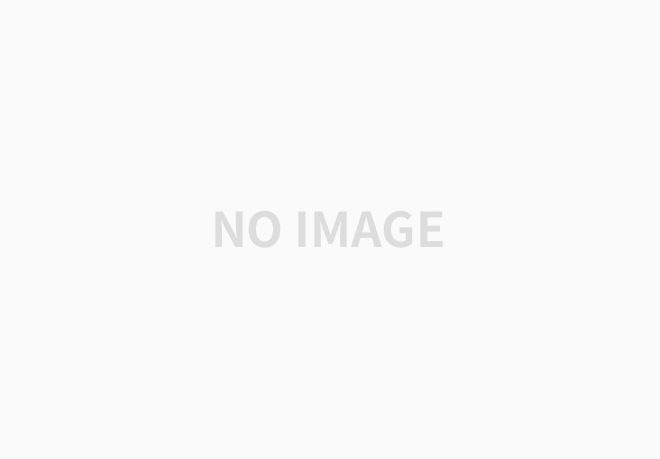
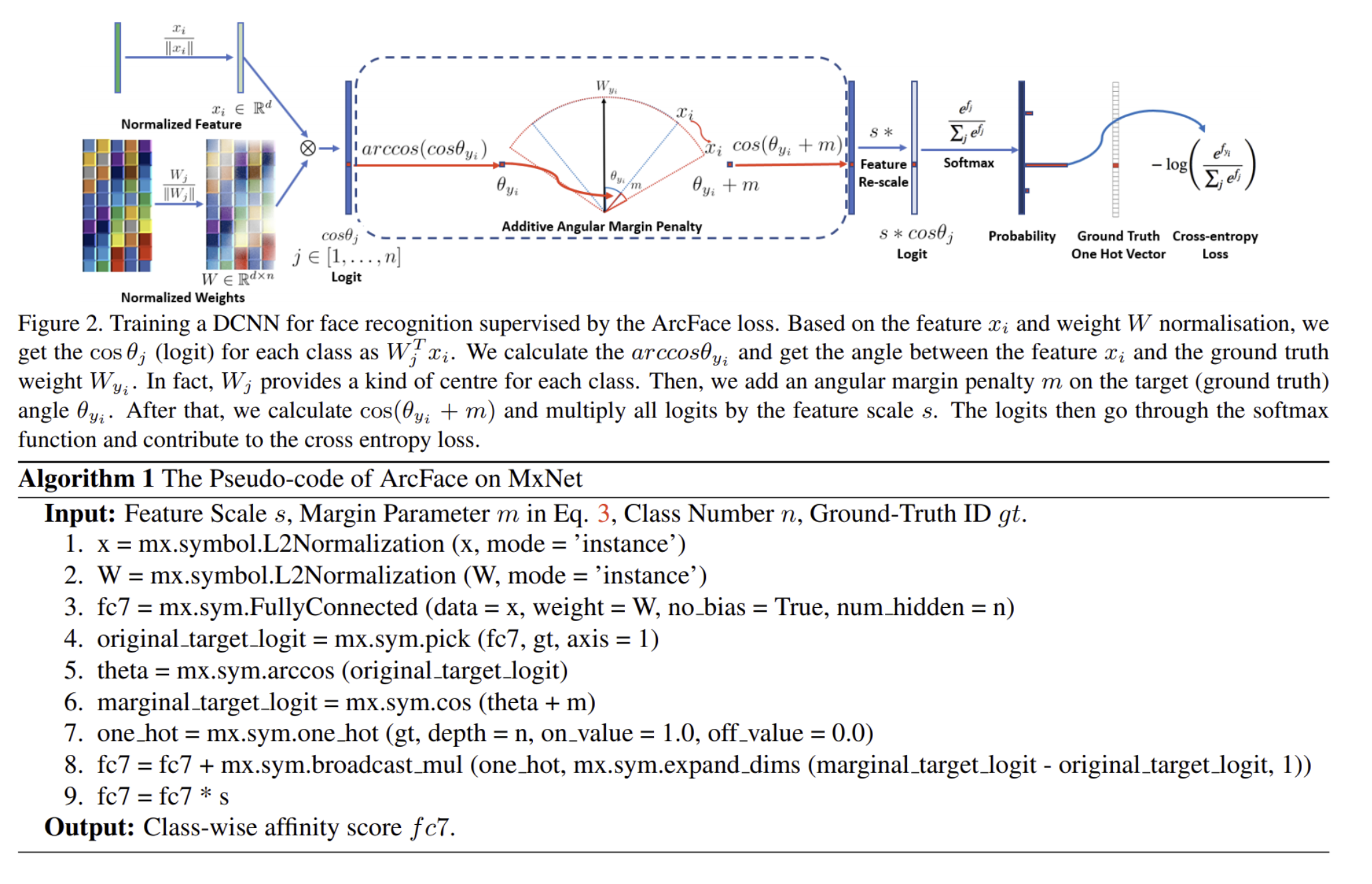
2. Proposed Approach
2.1. ArcFace
- 기존 softmax loss는 deep face recognition에서 널리 사용되나 feature embedding에 intra-class similarity와 inter-class diversity를 강제하지 않아 intra-class variation이 큰 경우 성능 차이가 심해진다.
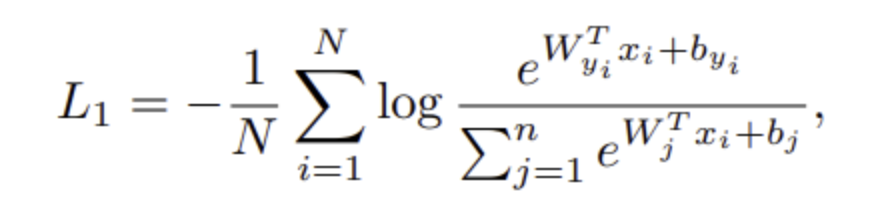
- simplicity를 위해 bias bj 를 0으로 고정
- logit을 WTjxi=||WJ||||xi||cosθj 로 설정, θj 는 W와 x사이의 각도
- ||Wj|| 를 L2 normalisation을 통해 1로 고정
- embedding feature ||xi|| 를 L2 normalisation 하고 s 로 rescaling
- Normalisation을 함으로써 prediction이 오직 x와 W사이의 angle에 의해 결정되도록 하였음
- 임베딩 features는 이제 radius s인 hypersphere에 분포하게 된다
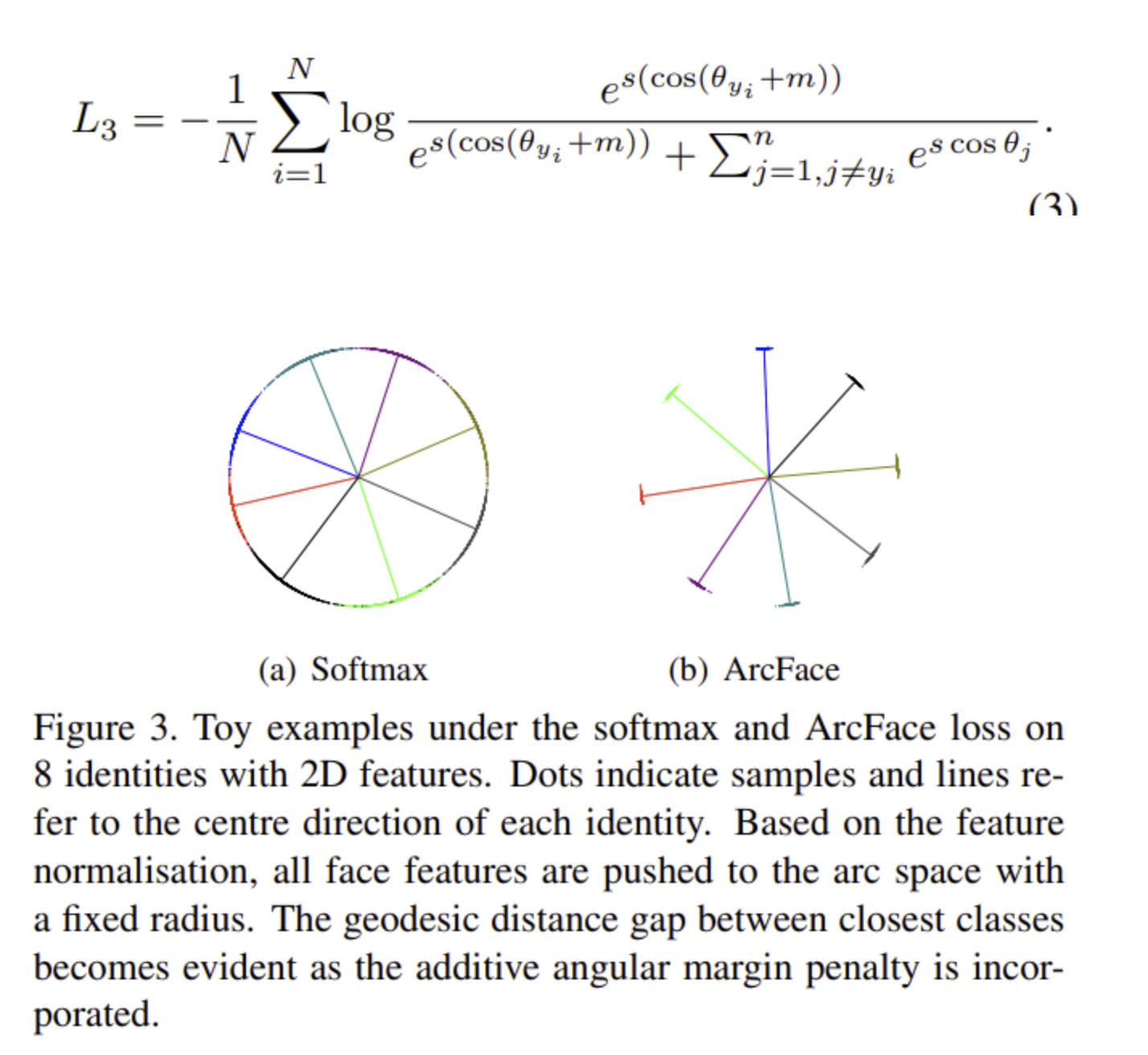
- Figure3이 ArcFace loss를 가장 쉽게 visualization 했다고 생각
- SphereFace의 margin
- 단점은 기하학적으로 해석이 어렵고 복잡한 식
- 단점은 기하학적으로 해석이 어렵고 복잡한 식
3. Experiments
- 대부분 Face datasets에서 좋은 성능
- face recognition domain-specific 한 내용이라 생략하였습니다.
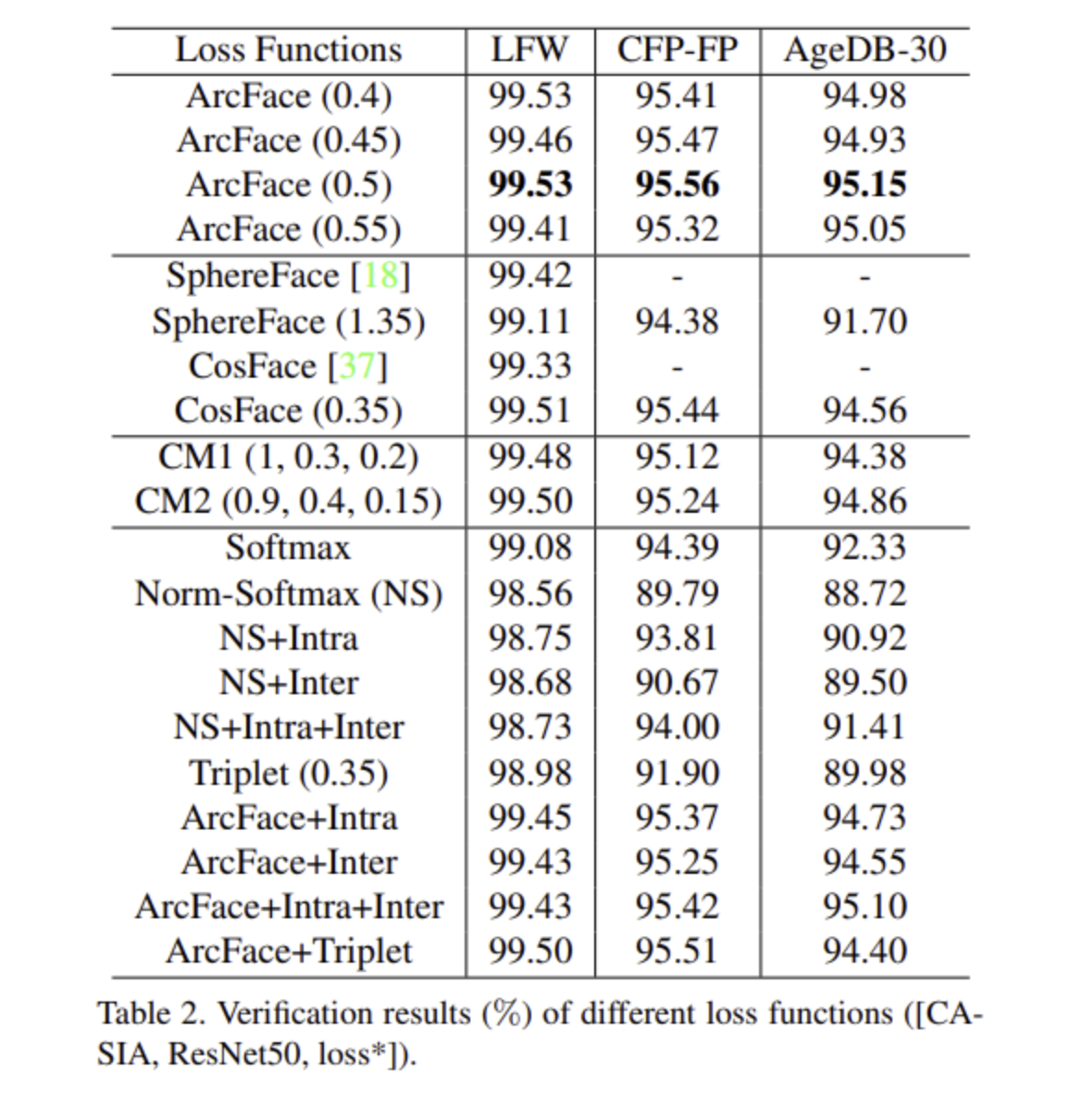
4. Conclusions
- face recognition에서 DCNN을 통해 학습된 피쳐 임베딩의 discriminative power를 향상시키는 Additive Angular Margin Loss function을 제시
- SOTA를 달성
후기 & 정리
- Face recognition task을 어렵게 하는 요인인 inter-class, intra-class feature embedding 분포 문제를 개선하기 위해 간단하고 쉽고 효과적인 ArcFace loss를 제시
- last FC layer의 weight matrix가 angular space에서 각 class의 centre가 될 수 있다는 SphereFace의 가정을 받아들여 사용
- Additive Angular Margin Penalty를 추가한 간단한 형태지만 SOTA를 달성
- SphereFace와 다르게 margin을 add하는 형식으로 Hypersphere에서의 Geodesic distance와 일치하게 되어 해석이 용이
- 각 class의 centre인 W를 학습하며 x와의 내적을 통해 구한 각도를 기반으로 softmax loss를 사용하여 해당 class의 W와 각이 최소가 되도록 학습 (θ 가 0이 될수록 cosine값은 1에 가까워짐)
- ArcFace를 통해 inter-class discrepancy와 intra-class compactness를 향상
- Metric Learning 방법론에 대한 이해를 높일 수 있던 논문
Reference
[0] Jiankang Deng and Jia Guo and Niannan Xue and Stefanos Zafeiriou. (2018). "ArcFace: Additive Angular Margin Loss for Deep Face Recognition". arXiv:1801.07698
[1] https://norman3.github.io/papers/docs/arcface.html
'AI > Deep Learning' 카테고리의 다른 글
Comments