일 | 월 | 화 | 수 | 목 | 금 | 토 |
---|---|---|---|---|---|---|
1 | 2 | 3 | 4 | 5 | ||
6 | 7 | 8 | 9 | 10 | 11 | 12 |
13 | 14 | 15 | 16 | 17 | 18 | 19 |
20 | 21 | 22 | 23 | 24 | 25 | 26 |
27 | 28 | 29 | 30 |
Tags
- 딥러닝
- Diffusion
- RNN
- animation retargeting
- userwidget
- cv
- WinAPI
- Generative Model
- multimodal
- 언리얼엔진
- BERT
- GAN
- 생성모델
- NLP
- deep learning
- Font Generation
- ue5.4
- 폰트생성
- Few-shot generation
- motion matching
- Unreal Engine
- Stat110
- 오블완
- UE5
- ddpm
- 디퓨전모델
- 모션매칭
- CNN
- dl
- WBP
Archives
- Today
- Total
Deeper Learning
CLIP: Learning Transferable Visual Models From Natural Language Supervision 본문
AI/Deep Learning
CLIP: Learning Transferable Visual Models From Natural Language Supervision
Dlaiml 2022. 11. 4. 20:07Alec Radford, Jong Wook Kim , Chris Hallacy, Aditya Ramesh, Gabriel Goh, Sandhini Agarwal, Girish Sastry, Amanda Askell, Pamela Mishkin, Jack Clark, Gretchen Krueger, Ilya Sutskever, (2021.02)
Abstract
- SOTA 컴퓨터 비전 시스템은 정해진 object의 카테고리를 예측하도록 학습하는 것
- 지도학습 방식은 라벨링된 데이터를 요구하기 때문에 일반화, 활용성에 제약이 존재
- raw text-image pair 데이터를 학습하면 지도학습에서 더 많은 소스를 활용할 수 있음
- raw text-image pair 데이터로 이미지 representation 학습을 위한 간단한 pre-training 태스크를 제시
- 제시한 pre-training 방식을 통해 학습된 visual concepts을 자연어를 통해 참고가 가능하며 downstream task에서 zero-shot transfer를 가능케 함
- 30개 이상의 컴퓨터 비전 데이터셋으로 다양한 task에서 성능을 측정 (OCR, action recognition, geo-localization, fine-grained object classification)
- ImageNet의 1.28M 학습 데이터를 사용하지 않고 해당 데이터로 학습한 ResNet-50과 견줄만한 성능의 모델을 학습시키는데 성공
- pre-trained model weights와 code를 공개 https://github.com/OpenAI/CLIP
GitHub - openai/CLIP: Contrastive Language-Image Pretraining
Contrastive Language-Image Pretraining. Contribute to openai/CLIP development by creating an account on GitHub.
github.com
1. Introduction and Motivating Work
- NLP에서는 raw text를 직접적으로 학습에 사용하는 여러 task-agnostic objectives(autoregressive, masked language modeling)가 연구되었음
- 하지만 CV(Computer vision)에서는 ImageNet과 같은 crowd-labeled 데이터셋에서 학습하는 방식이 주류
- image caption을 사용하여 효율적인 이미지 representation을 학습하려는 연구가 활발하지는 않지만 몇몇 있었음(Visual N-Grams)
- 하지만 다른 대안들보다 대체로 낮은 성능을 기록
- Visual N-grams 11.5% zero shot accuracy on ImageNet
- 최근에는 VirTex, ICMLM, ConVIRT와 같은 연구들이 transformer-based language modeling, masked language modeling, contrastive objectives의 가능성을 보여줌
- 지금까지 연구 방식은 제한된 데이터로 지도학습 하는 전통적인 방식과 raw text에서 바로 학습하는 방식의 중간에 위치한 방법론들
- 지도 학습 방식, static softmax classifier의 사용이 zero-shot 성능과 flexibility를 제한함
- large scale로 자연어를 활용하여 image classifier를 학습하는 ConVIRT의 simplified version인 CLIP(Contrastive Language-Image Pre-training)을 제시
- CLIP은 GPT와 같이 다양한 downstream task에서 좋은 성능을 기록
- task-specific supervised 모델에 견줄만한 성능을 CLIP을 활용한 zero-shot transfer로 달성
- CLIP은 공개된 가장 뛰어난 ImageNet 학습 모델보다 뛰어난 성능과 computational efficency를 달성
- 비슷한 accuracy를 가진 ImageNet 지도학습 모델보다 robust하여 zero-shot에 적합한 task-agnostic 모델
2. Approach
2.1. Natural Language Supervision
- CLIP의 핵심 아이디어는 자연어(caption)에 내포된 perception을 지도학습 방식으로 학습하는 것
- 자연어에 내포된 image representation을 학습하는 것은 새로 제시된 아이디어가 아닌 이전부터 관점에 따라 다양한 용어들로 표현되던 방식 (supervised, self-supervised, unsupervised)
- 본 논문에서는 이를 Natural Language Supervision으로 명명
- 자연어를 사용한 학습은 기존 crowd-sourced labeling image 데이터보다 인터넷에서 데이터를 쉽게 구할 수 있어 scale 측면에서 장점
- image representation 학습뿐만 아니라 이를 자연어와 연결한다는 점에서 flexible zero-shot transfer가 가능
2.2. Creating a Sufficiently Large Dataset
- 인터넷에 공개된 소스로 400M의 image-text pair 데이터셋을 구성
2.3. Selecting an Efficient Pre-Training Method
- large scale의 자연어 지도학습을 위해 training efficiency는 매우 중요함
- 첫 시도로는 VirTex와 비슷하게 image CNN과 text transformer를 사용하여 image의 caption을 예측
- Figure 2를 보면 BoW prediction에 비해 효율이 크게 떨어짐
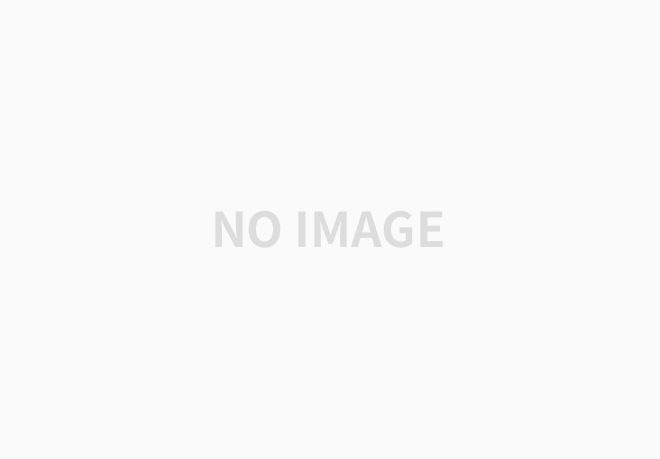
- contrastive objectives가 더 좋은 image representation을 적은 계산량으로 학습할 수 있다는 이전 연구 결과에 따라 어떤 text가 어떤 image와 매칭되는지를 학습
- N개의 image, text pairs 데이터에서 CLIP은 N x N개의 가능한 pairing에 대한 예측값을 계산
- CLIP 은 image encoder와 text encoder를 real pairs의 cosine similarity를 최대화하는 방식으로 학습하여 multi-modal embedding space를 학습
- deep metric learning 분야의 연구인 multi-class N-pair loss에 제시하였던 학습방식
- Figure 2에서 볼 수 있듯이 4배 더 효율적인 계산량
- 학습 방식은 아래 Figure 1, Pseudo code 참고
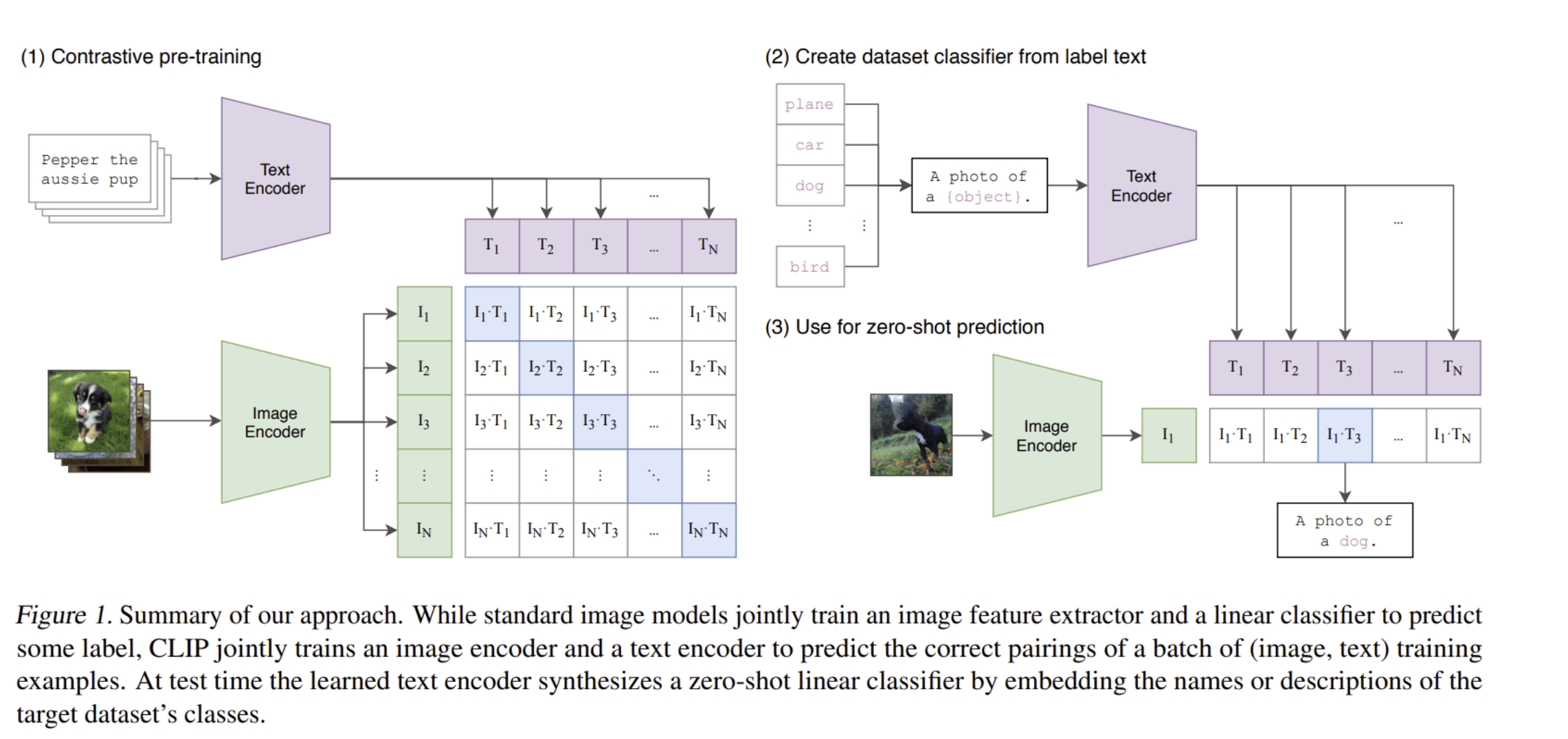
# image_encoder - ResNet or Vision Transformer
# text_encoder - CBOW or Text Transformer
# I[n, h, w, c] - minibatch of aligned images
# T[n, l] - minibatch of aligned texts
# W_i[d_i, d_e] - learned proj of image to embed
# W_t[d_t, d_e] - learned proj of text to embed
# t - learned temperature parameter
# extract feature representations of each modality
I_f = image_encoder(I) #[n, d_i]
T_f = text_encoder(T) #[n, d_t]
# joint multimodal embedding [n, d_e]
I_e = l2_normalize(np.dot(I_f, W_i), axis=1)
T_e = l2_normalize(np.dot(T_f, W_t), axis=1)
# scaled pairwise cosine similarities [n, n]
logits = np.dot(I_e, T_e.T) * np.exp(t)
# symmetric loss function
labels = np.arange(n)
loss_i = cross_entropy_loss(logits, labels, axis=0)
loss_t = cross_entropy_loss(logits, labels, axis=1)
loss = (loss_i + loss_t)/2
- 이전에 제시된 contrastive 방법과 다르게 representation과 contrastive embedding space 사이에 non-linear projection을 하지 않음 (pseudo code에서 보면 W와 dot 연산이 전부인 linear projection)
- image random crop, single sentence 추출도 하지 않음
2.4. Choosing and Scaling a Model
- Image encoder로 ResNet-50(ResNet-D)에 antialised rect-2 blur pooling(Richard Zhang, 2019)을 적용한 모델과 ViT를 사용
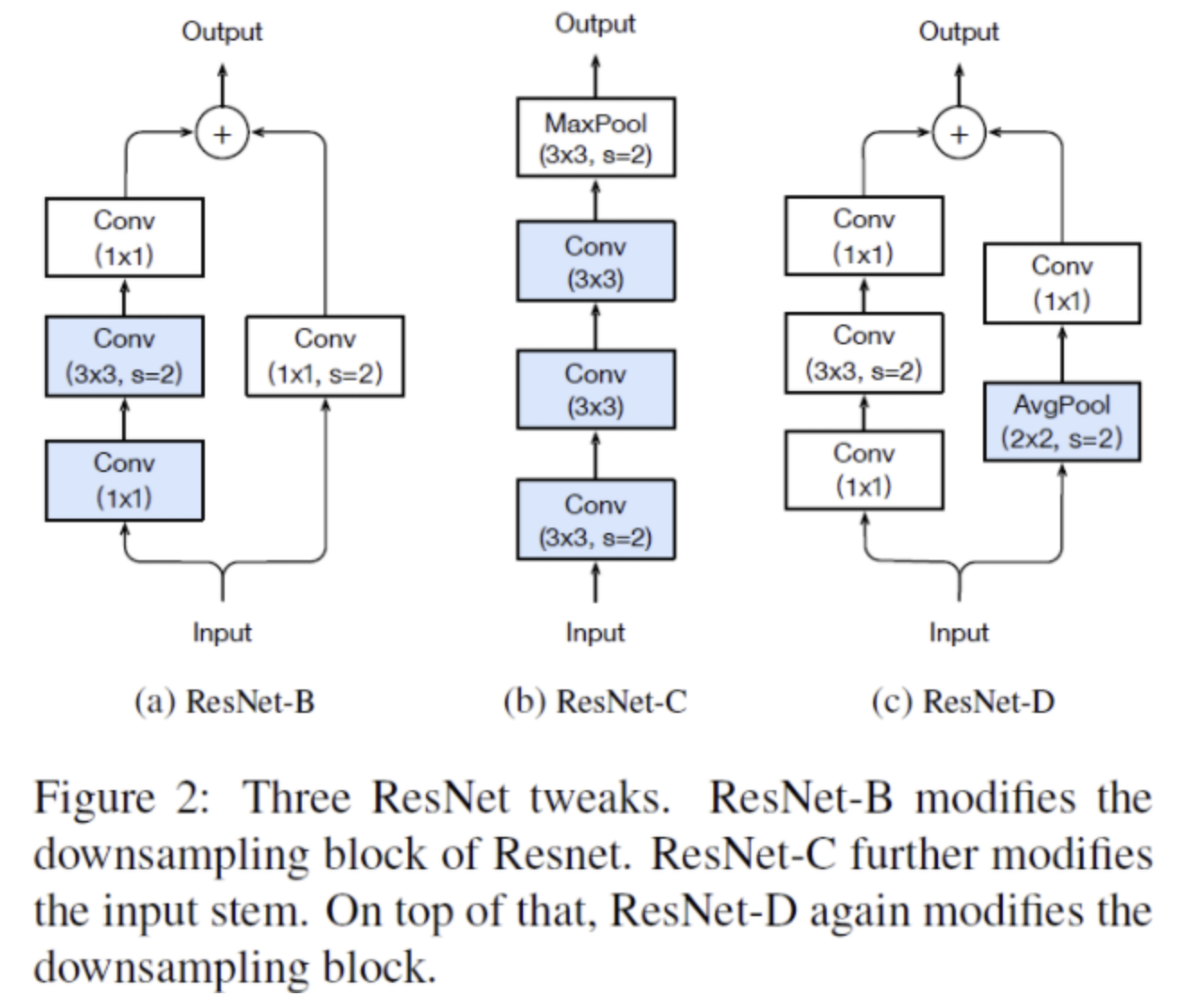
- ResNet Image encoder의 global average pooling layer를 attention pooling으로 변경
- query가 global average-pooled representation에 의해 conditioned된 transformer-style의 multi-head QKV attention
- Transformer Image encoder는 ViT의 설정을 거의 따랐으며 initialization 방식 변경, layer normalization 추가 정도의 수정만 하였음
- Text encoder는 GPT-2 버전의 Transformer를 사용
- max sequence length를 76으로 설정
- masked self-attention 사용
- Text Encoder에서는 width를 scale, Image Encoder에서는 width, depth, resolution을 같은 비율로 연산량이 증가하도록 scale
- text encoder의 성능은 CLIP의 성능에 적은 영향을 미치는 것을 확인
2.5. Training
- ResNet-50,101 기반으로 EfficientNet-style로 scaling한 4x, 16x, 64x 연산량이 추가된 모델로 학습 (RN50x4, RN50x16, RN50x64)
- ViT-B/32, ViT-B/16, ViT-L/14 학습. 모든 모델은 32 epochs 학습
- Adam optimizer (decoupled weight decay regularization 사용)
- softmax의 logits의 범위를 조절하는 학습되는 temperature parameter τ 는 0.07로 initialize
- logits이 100이상으로 커지지 않도록 cliping 하는 것이 중요함
- batch size: 32768, mixed-precision 사용
- 가장 큰 image encoder는 592 V100 Gpus에서 18일 학습, 가장 큰 text encoder는 256 V100 gpus에서 12일 학습
3. Experiments
3.1. Zero-Shot Transfer
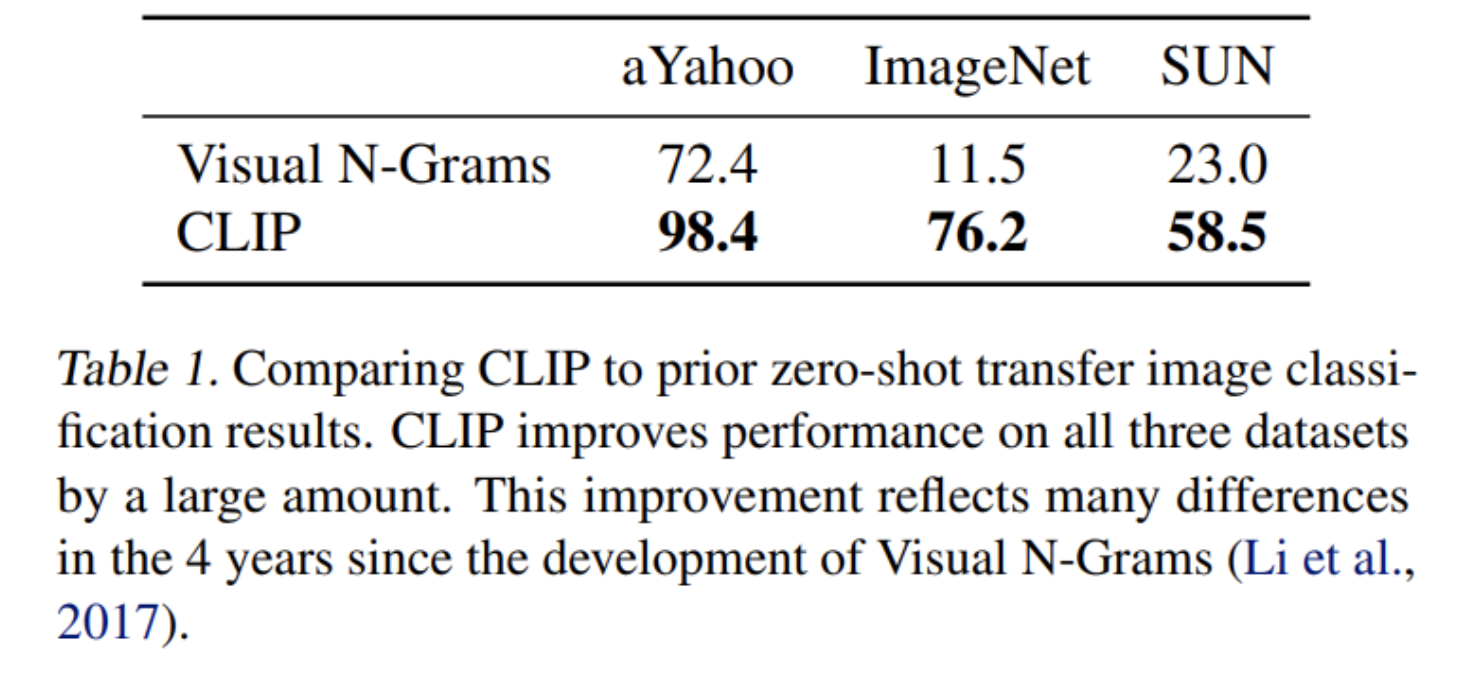
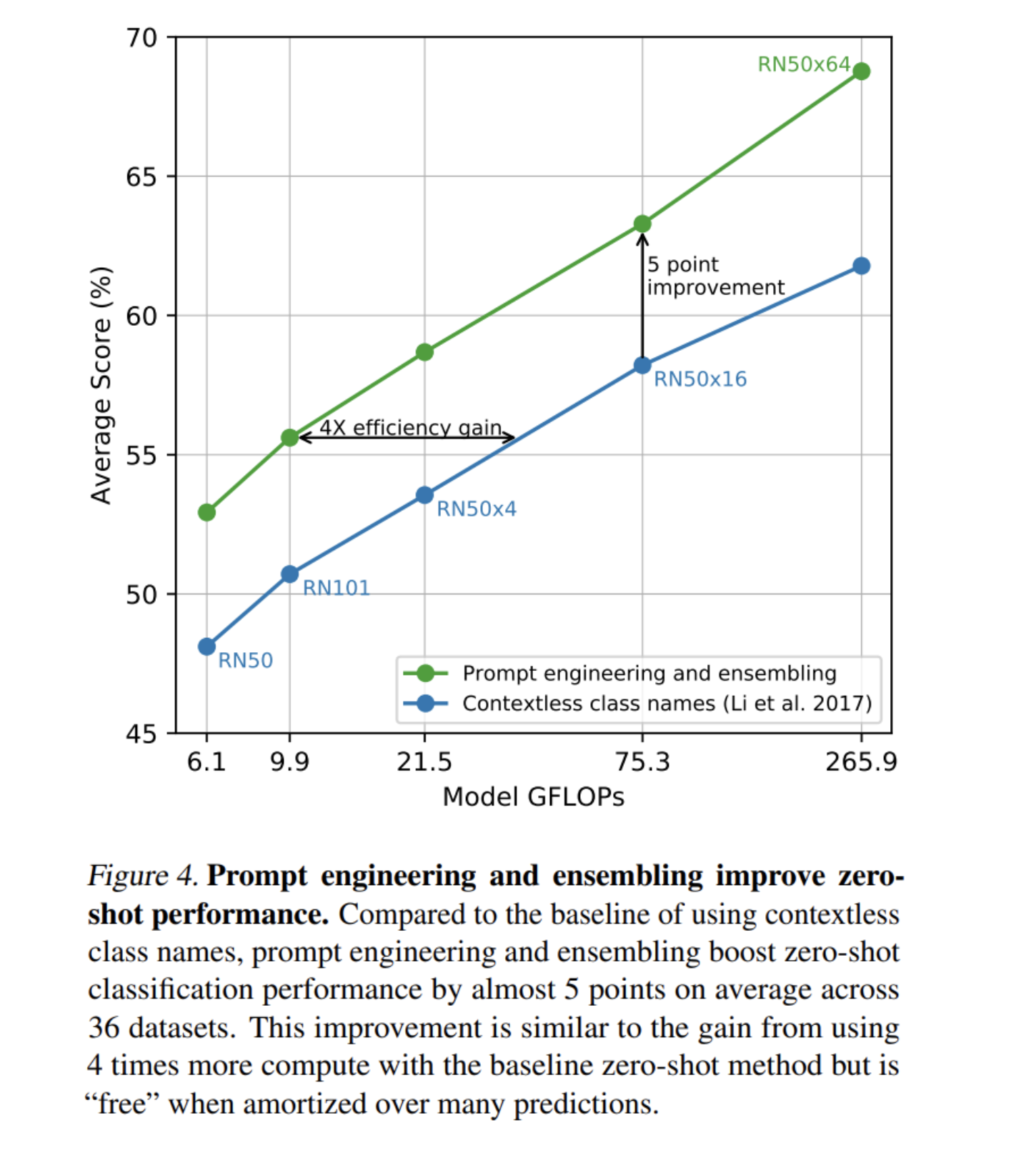
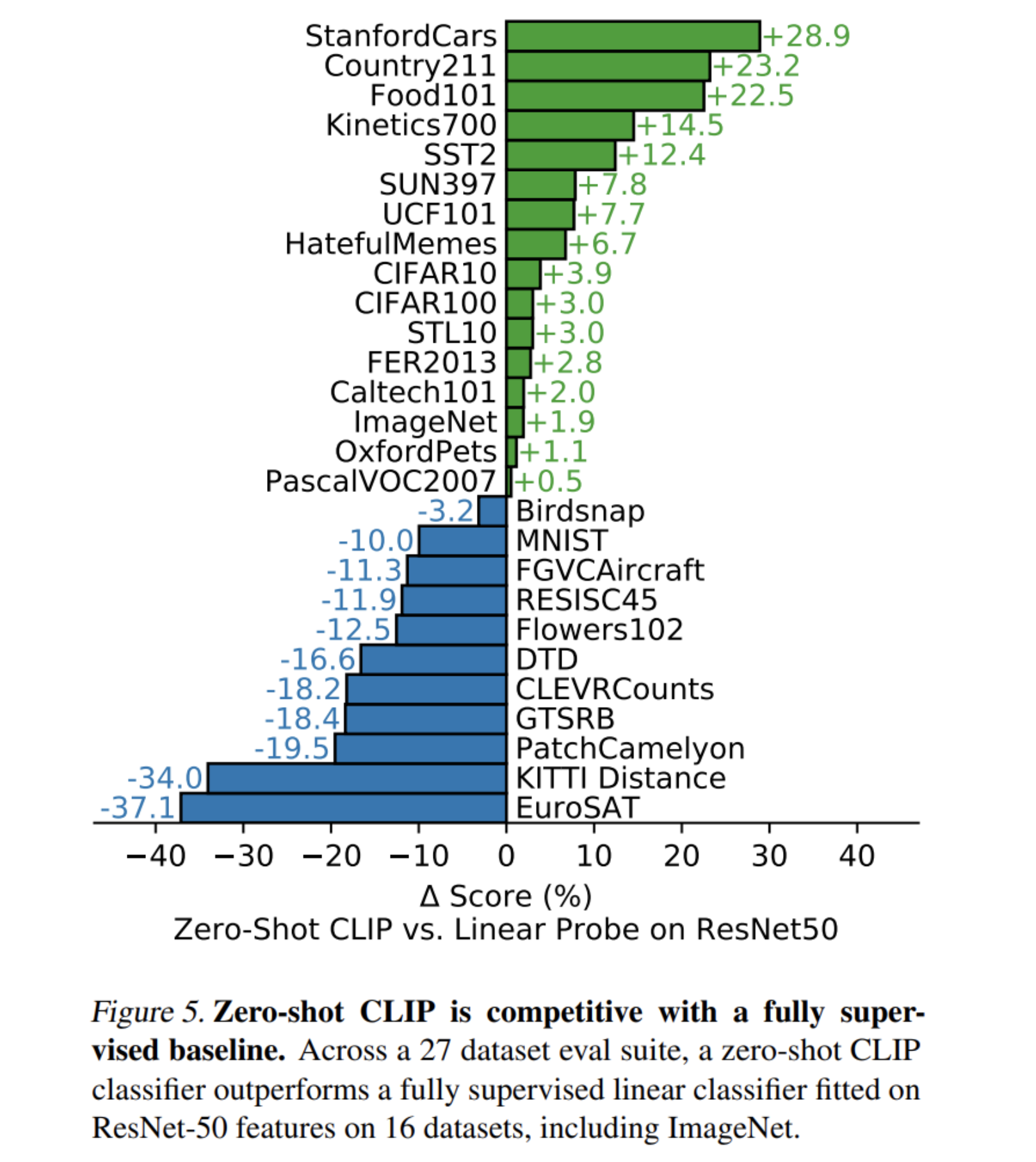
- classification에서 visual n-gram보다 뛰어난 성능
- “A photo of a {label}”, “A photo of a {label}, a type of pet” 등의 task에 알맞은 prompt engineering과 “A photo of a big {label}”, “A photo of a small {label}” 등 prompt를 사용하고 embedding space에서 ensemble하여 성능 향상
- 추상적이고 특수한 dataset을 사용하는 경우 fully supervised baseline에 비해 성능이 저하
- 다른 few-shot 모델보다 CLIP의 zero-shot 성능이 더 뛰어났음
3.2. Representation Learning
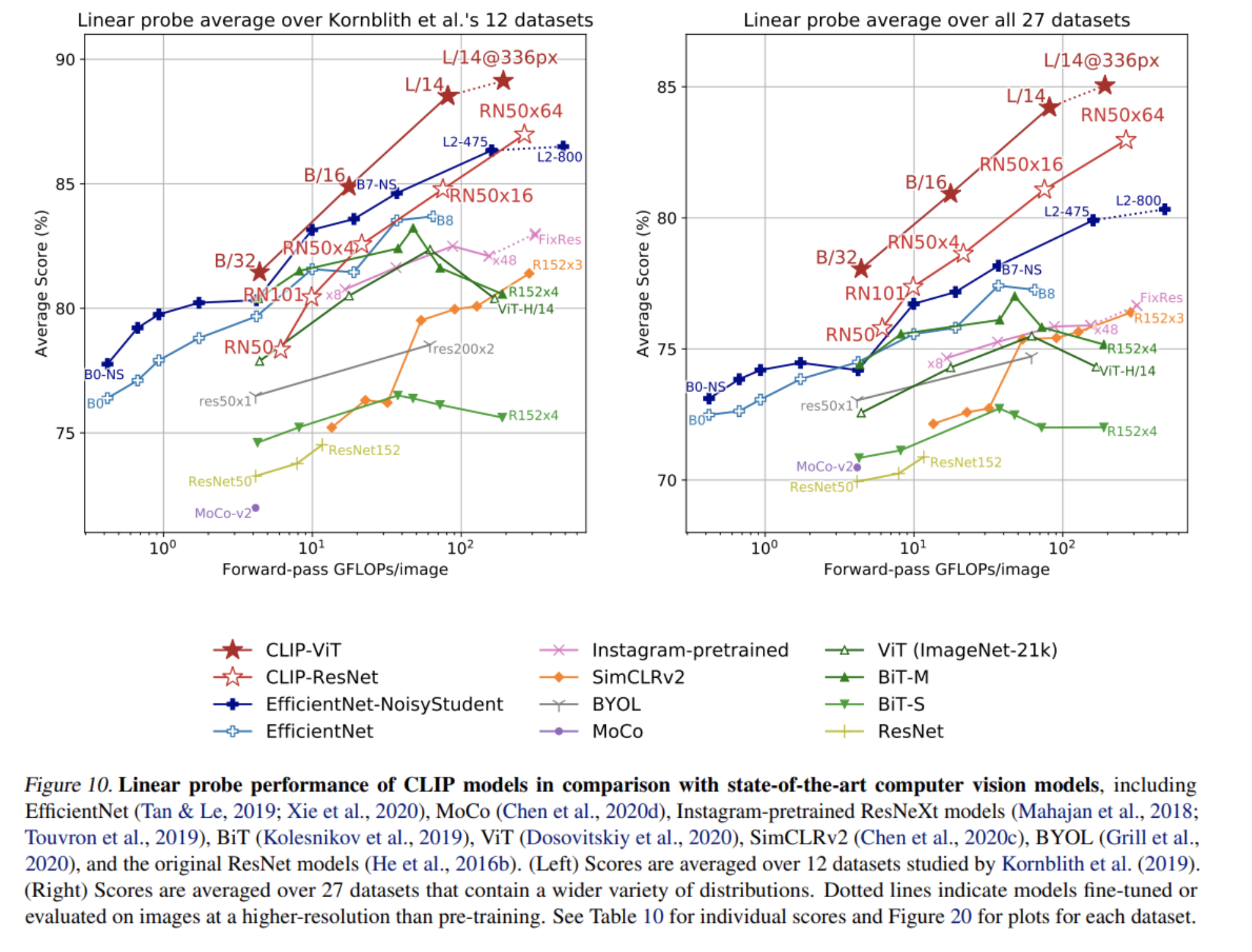
- CLIP의 representation을 사용하여 classifier를 학습시킨 결과
- CLIP은 best ImageNet 모델이 학습한 representation보다 좋은 representation을 학습
3.3. Robustness to Natural Distribution Shift
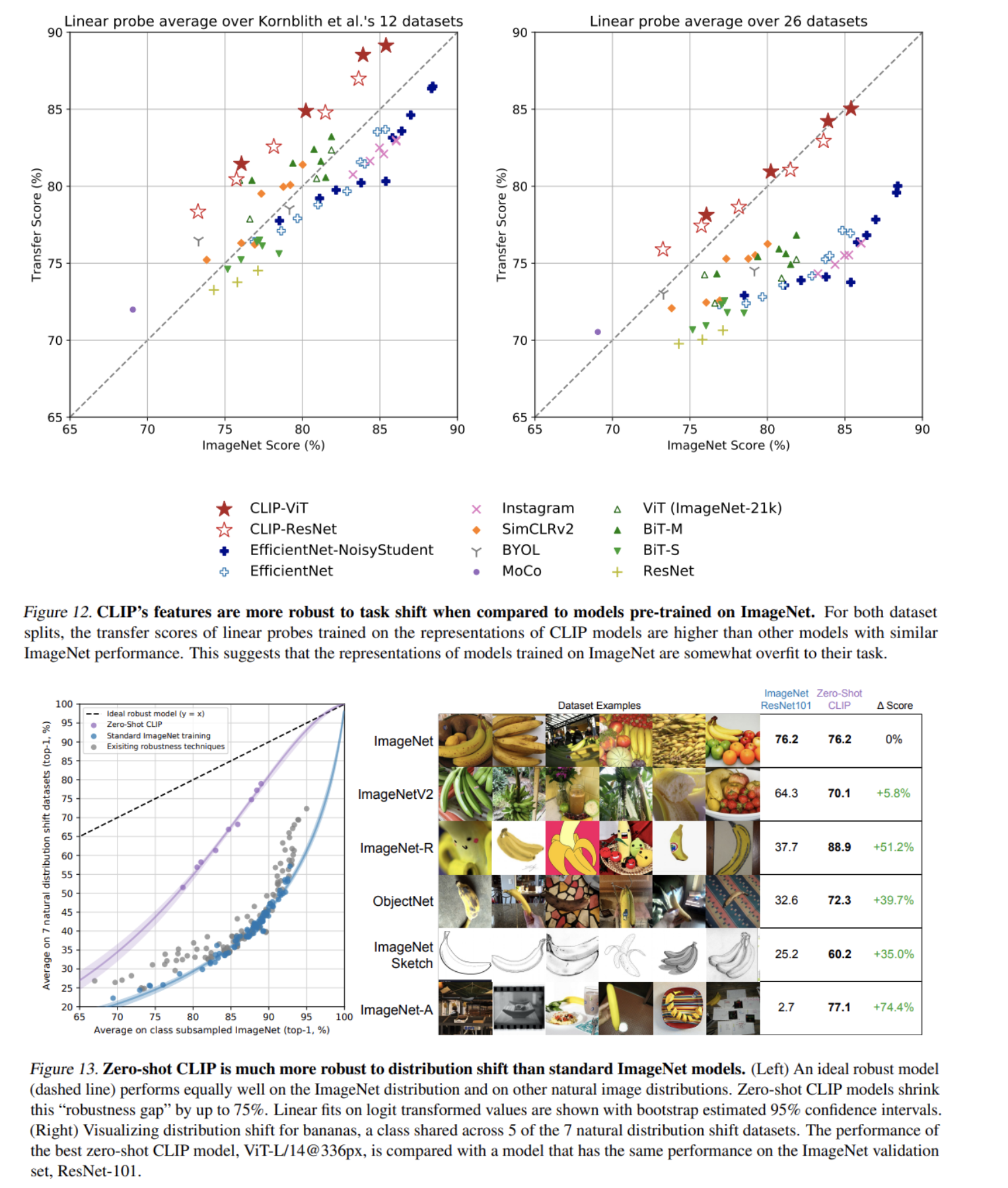
- ImageNet 학습 모델보다 Distribution Shift에 훨씬 robust한 것을 볼 수 있음
- ImageNet 데이터로 추가 학습하여 adaptation을 하면 CLIP도 robustness를 잃지만 해당 추가 학습 데이터에서 성능은 증가
4. Comparison to Human Performance
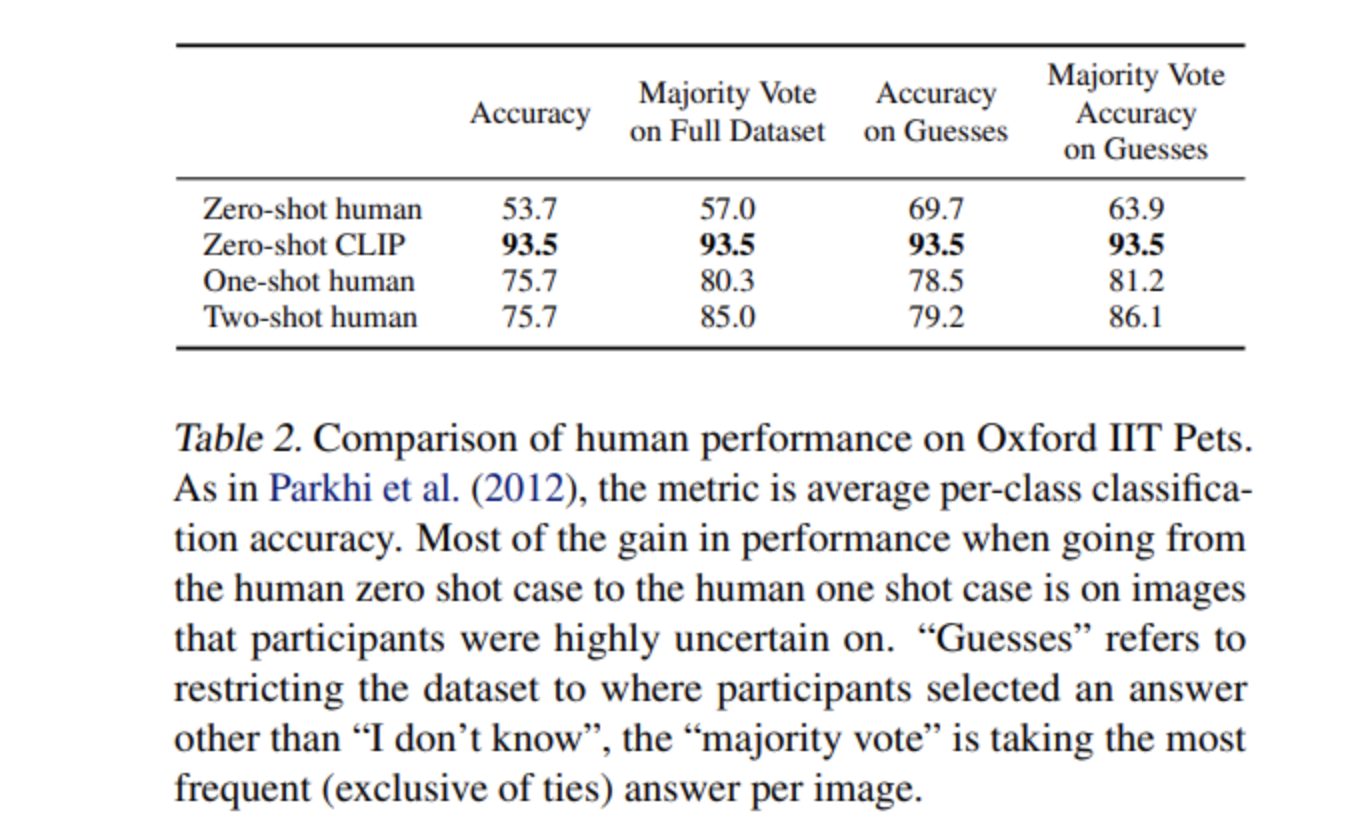
- Oxford IIT Pets 데이터에서 Human Performance와 비교. Zero-shot에서 CLIP이 더 뛰어나다고 저자는 말함
5. Data Overlap Analysis
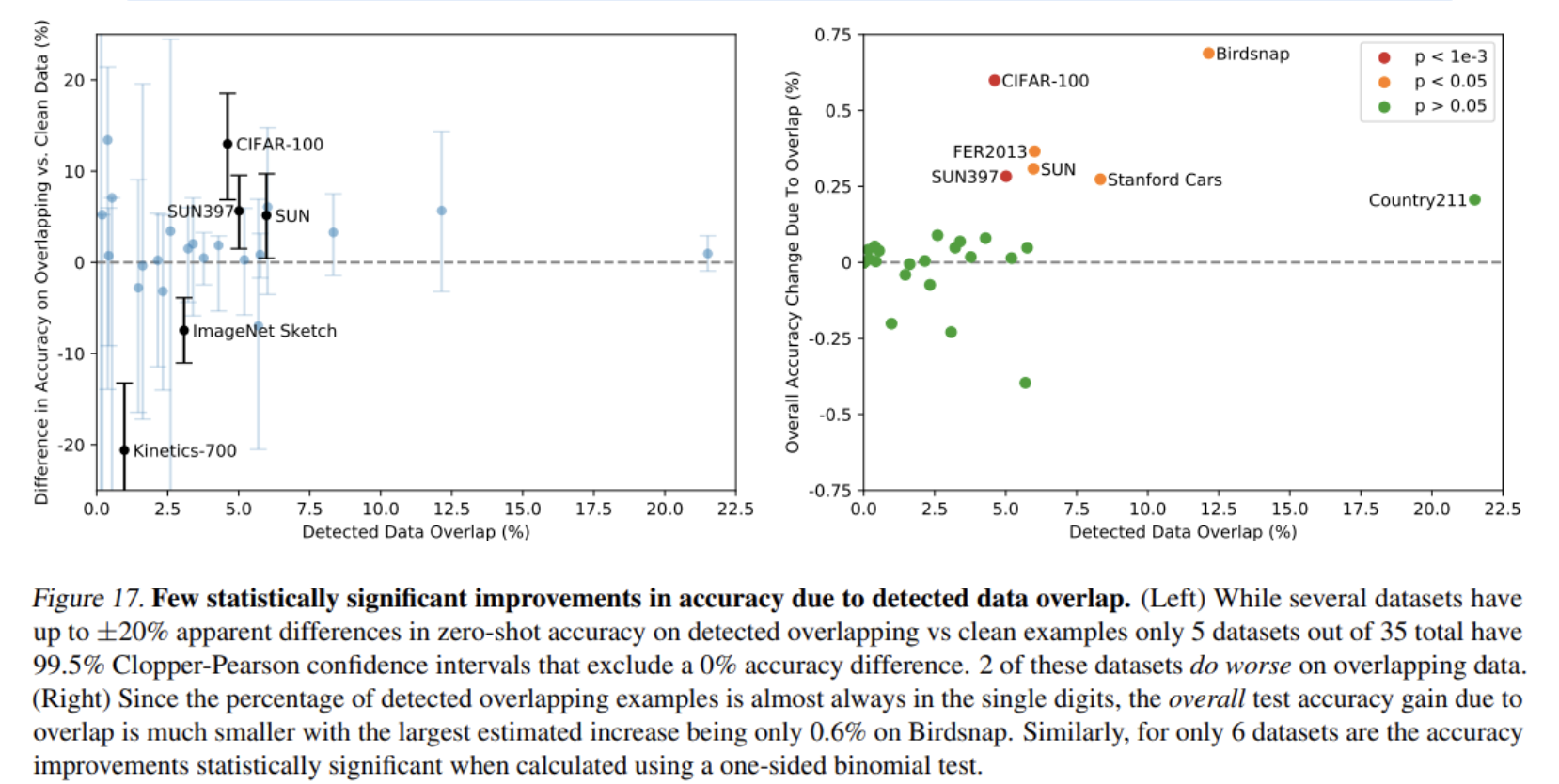
- 인터넷에서 얻은 매우 큰 데이터셋을 사용하다 보니 의도하지 않은 data overlap이 downstream task에서 발생 가능
- 데이터셋마다 얼마나 Overlap이 발생하였는지, 발생한 Overlap으로 성능이 얼마나 부풀려졌는지 측정
- 6개 데이터셋이 1% 미만의 작은 cheating이 있었음
6. Limitations
- 아직 CLIP의 zero-shot 성능은 ResNet-50의 supervised baseline 수준이며 이는 현재 SOTA에 비해 부족
- 저자는 1000배의 계산량이 더해지면 SOTA와 경쟁 가능한 성능이 나올 것이라고 생각, 따라서 연산 효율성 증대가 필요
- fine-grained classification (자동차 모델, 꽃 종류) 등 task에 취약하며 가장 가까운 자동차의 거리를 구하는 것과 같은 새로운 task에 매우 취약
- MNIST에서의 성능도 단순한 logistic regression baseline과 비슷한데 이는 사전 학습 dataset에 숫자 데이터가 적어서 발생한 성능 문제며 이는 딥러닝 모델의 고질적인 문제인 generalization을 해결하지 못하였다는 것을 말함
7. Broader Impacts
- 성별에 따른 Bias, 악용 가능성이 존재
- 인터넷 데이터에 존재하는 Bias를 그대로 학습하기 때문에 개선이 필요
8. Conclusion
- NLP에서의 task-agnostic web-scale pre-training을 다른 domain으로 transfer
- CLIP의 학습 objective를 optimize하기 위해 모델은 학습 과정 중에 다양한 task 수행능력을 학습
- 자연어 prompt를 통해 학습한 task 수행능력을 활용할 수 있으며 기존 데이터셋에서 좋은 zero-shot 성능을 보임
- CLIP은 충분한 scale을 갖추면 task-specific supervised model과도 경쟁할 수 있는 모델이며 개선할 부분도 많음
후기 & 정리
- 기존 NLP에서의 web-scale task-agnostic pre-training을 image data에 함께 적용한 새로운 방식을 제시
- contrastive 학습 방식을 통해 large caption dataset에 내재된 image에 대한 좋은 representation을 학습하여 뛰어난 zero-shot 성능을 보임
- 연산 효율, 학습방식, 활용 등을 future work으로 제시
Reference
[0] Richard Zhang. (2019). “Making Convolutional Networks Shift-Invariant Again”. https://arxiv.org/pdf/1904.11486.pdf
[1] Alec Radford et al. (2021). "Learning Transferable Visual Models From Natural Language Supervision”. https://arxiv.org/abs/2103.00020
'AI > Deep Learning' 카테고리의 다른 글
Comments