일 | 월 | 화 | 수 | 목 | 금 | 토 |
---|---|---|---|---|---|---|
1 | 2 | 3 | 4 | 5 | ||
6 | 7 | 8 | 9 | 10 | 11 | 12 |
13 | 14 | 15 | 16 | 17 | 18 | 19 |
20 | 21 | 22 | 23 | 24 | 25 | 26 |
27 | 28 | 29 | 30 |
- NLP
- WBP
- Font Generation
- ddpm
- Stat110
- Unreal Engine
- motion matching
- CNN
- UE5
- ue5.4
- cv
- GAN
- 오블완
- 폰트생성
- Few-shot generation
- WinAPI
- 생성모델
- dl
- 언리얼엔진
- RNN
- userwidget
- BERT
- animation retargeting
- 디퓨전모델
- 모션매칭
- deep learning
- multimodal
- Generative Model
- 딥러닝
- Diffusion
- Today
- Total
목록전체 글 (220)
Deeper Learning
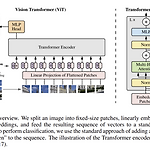
Alexey Dosovitskiy et al., (2020), Google Research, Brain Team Abstract 사실상 Transformer 구조가 NLP task에서 standard가 되었지만 vision task에서는 아직 적용에 한계가 있었음 Transformer는 CNN을 대체하지 못하고 CNN의 일부 컴포넌트를 대체하는 식으로 결합하여 사용되고 있었음 이미지 분류 태스크에서 pure transformer로 좋은 성능을 낼 수 있음 large datasets에서 pre-trained 한 ViT 모델은 mid-sized or small image recognition(ImageNet, CIFAR-100, VTAB, etc)에서 더 적은 computational cost를 필요로 하면..
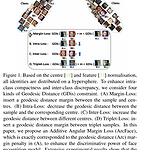
2018, Stefanos Zafeiriou Imperial College London Jiankang Deng et al. Abstract Deep Convolutional Neural Networks를 사용하여 large-scale face recognition에서 feature learning을 할 때 challenge는 discriminative power를 향상시키기 위한 loss function 설계 Centre loss는 deep features와 해당 class의 centre 사이의 Euclidean space에서 distance에 penalty를 주어 intra-class compactness를 달성 Sphere Face는 last fc layer의 linear transformation..
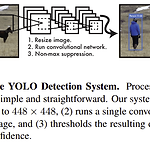
2016, University of Washington, Allen Institute for AI, Facebook AI Research source: https://arxiv.org/pdf/1506.02640.pdf Abstract YOLO, obejct detection을 위한 새로운 method를 제시 이전 연구들은 classifier를 detection을 위해 사용하였으나 YOLO는 object detection을 spatially separated bbox와 해당하는 bbox의 class probabilities를 예측하는 regression 문제로 정의 하나의 신경망 모델이 full image에서 one-stage로 bbox와 class probabilities를 예측 전체 detection ..
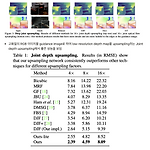
Abstract Convolutions은 CNN의 기본 building block spatially shared weights는 CNN이 쓰이는 이유임과 동시에 한계점이다 learnable local pixel features에 따라 변하는 pixel-adaptive convolution(PAC)를 제시 PAC는 자주 쓰이는 filter들의 일반화 버전으로 많은 case에서 그대로 사용이 가능하다. deep join image upsampling에서 SOTA fully-connected CRF를 PAC-CRF로 대체하여 성능, 속도 향상 pre-trained networks에서 PAC drop-in replacement로 성능 향상 1. Introduction standard convolution의 두 ..
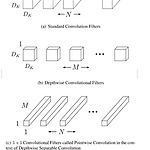
Abstract mobile, embedded vision application을 위한 depth-wise seperable convolutions을 활용한 경량화 모델 MobileNets을 제시 latency와 accuracy의 trade off를 조정하는 2개의 global hyperparameters 제시 MobileNets을 다른 모델과 비교하며 여러 실험을 통해 효율성을 검증하였음 다양한 task에 적용 가능 1. Introduction limited platform에서 빠르게 동작하여야 하는 real-word의 application이 다수 존재 (Robotics, self-driving) 2개의 hyper-parameters를 사용하여 모바일 및 embedded vision applicatio..
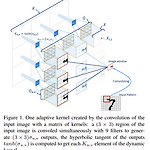
Abstract CV recognition performance을 향상시키기 위해 많은 복잡한 neural network architectures가 연구되었다. high computing resource를 요구하지 않고 온 디바이스로 한정된 resource에서 모델을 동작시키기 위해 adaptive convolutional kernel을 제시 input image가 dynamic하게 kernel을 결정 Adaptive kernels을 사용한 모델은 기존 CNN 보다 2배 이상 수렴이 빨랐으며, LeNet base model에서 MNIST dataset에 대해 99% 이상의 정확도를 달성하는데 66배 적은 parameters만 필요로 하였음 1. Introduction SOTA CV 모델은 대부분 deep..
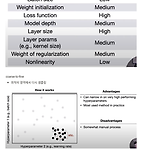
Lecture 5Setting up ML Projects85% of AI projects fail Prioritizing projectsML project의 feasibilityData availabilitystable?hard to acquire?expensive labeling?how much data is needed?security requirements?Accuracy requirementhow costly are wrong pred?how frequently does the system need to be right to be useful?ethical implicationsProblem difficultyis the problem well-defined?good published work o..
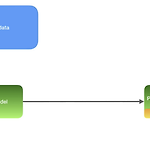
Lecture 4 Transfer Learning 10k labeled 데이터로 classifier 학습. 이미지넷처럼 ResNet-50을 사용하고 싶으나 모델이 너무 커 작은 데이터에 쉽게 오버피팅 ImageNet에서 pretrained된 ResNet-50을 load하고 10k data로 fine-tuning → 성능 향상 Language Model need to converting word to vectors one hot encoding Scaled poorly with vocab size Very high-dimensional sparse vectors → NN operations work poorly Violates what we know about word similarity (ex. dist..
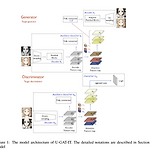
Abstract end-to-end로 학습 가능한 normalization function과 어텐션 모듈을 사용한 unsupervised image-to-image translation method를 제시 어텐션 모듈은 auxiliary classifier에 의해 얻은 attention map을 사용하여 중요한 region에 모델이 집중하도록 guide 이전 attention-based method와 다르게 두 도메인 간 geometric change가 가능 dataset에서 얻은 paramters로 shape와 texture 변화량을 flexible 하게 변화시킬 수 있는 AdaLIN(Adaptive Layer-Instance Normalization) 기존 SOTA보다 좋은 퀄리티 1. Introdu..
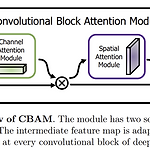
Abstract intermediate feature map에서 순차적으로 channel, spatial attention을 적용하는 모듈을 제시 weight 수가 적으며 모든 CNN 아키텍처에 쉽게 적용할 수 있는 일반적인 모듈 ImageNet-1K, MS COCO detection, VOC 2007 detection dataset에서 성능 향상이 있었음 1. Introduction CNN은 vision task에서 rich representation power를 바탕으로 좋은 성능을 보였다. CNN의 성능을 향상시키기 위해 최근 Depth, Width, Cardinality에 대한 연구가 주로 진행되고 있다. 어텐션은 어디에 집중해야 하는지 말해주는 것만이 아니라 중요한 영역에 대한 represent..
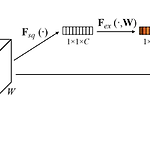
Abstract CNN의 메인 블록은 spatial, channel-wise information를 각 layer의 local receptive fields에서 결합하여 정보를 가지고 있는 feature를 만든다. 이전 연구들은 spatial encoding을 강화하는데 집중하였지만 저자는 channel relationship에 집중하여 "Squeeze-and-Excitation"(SE) block을 제시 SE block은 adaptive 하게 channel-wise feature를 recalibrate(재교정, 재보정)한다. computational cost가 크지 않으며 ILSVRC에서 1등을 차지 1. Introduction CNN은 필터들은 spatial, channel-wise 정보를 함께 사용..
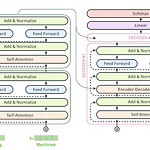
Transformer 트랜스포머 모델은 기존의 seq2seq 모델에서 Encoder, Decoder 형태를 유지하면서 RNN을 사용하지 않고 어텐션 스코어를 중심으로 학습을 하는 모델이다. 기존 Attention 모델에서 seq2seq의 Encoder가 Decoder로 정보를 전달할 때 hidden state에 정보를 모두 담기가 어렵고 시점에 따른 정보 희석의 문제를 해결하기 위해 어텐션 스코어를 사용하여 이를 보정하였다면 트랜스포머 모델은 어텐션 스코어 자체를 Encoder와 Decoder사이의 연결점으로 사용한다. Multi-head-Self-Attention 트랜스포머 모델은 셀프 어텐션을 통해 계산한 어텐션 스코어를 사용하기 때문에 먼저 셀프 어텐션에 대해 알아보겠다. 셀프 어텐션은 한 문장에서..
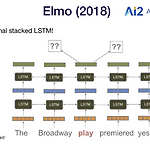
Beyond Embeddings Word2Vec, Glove 임베딩은 13~14년에 인기 많은 task에서 좋은 성능을 보였음 Problem: representations are shallow 첫 번째 layer만 all Wikipedia data로 pretrained한 embedding의 이점을 가짐 나머지 layer (LSTMs)는 pretrained data를 보지 못하고 own data로만 학습됨 Bank Account와 River Bank에서 Bank는 전혀 다른 의미를 가지지만 Word2Vec 또는 Glove로는 이를 반영하지 못하고 Bank가 같은 벡터로 임베딩 되어 사용됨 문맥을 반영한 워드 임베딩 (Contextualized Word Embedding)이 필요하다. → embedding ..
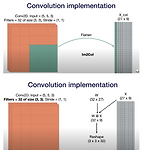
개괄적인 정리 for 그룹스터디 발표 Lecture 2.A Convolutional Networks Fc layer를 사용하지 않는 이유 연산량 image가 움직였을 때 모든 input의 위치가 변함 ( translation variant ) filter math W' = floor((W-F+2P) / S + 1) half padding == same padding full padding: 3x3 filter 기준 (2,2) padding, image가 1 pixel이라도 kernel에 포함되도록 하는 최대 패딩 (3,3) padding을 할 경우 kernel이 padding으로만 이루어진 영역을 계산함. (의미 없음) Transposed convolution Upsampling을 위한 기법 conv는 ..
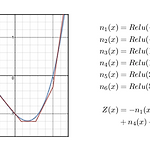
Universal Approximation Theroem one hidden layer로 모든 함수를 근사할 수 있다는 이론. 신경망은 함수를 근사하는데 매우 뛰어난 method로 여러 신경망 층을 쌓은 딥러닝이 비정형 데이터에서 최고의 성능을 보이고 있다. 하지만 one hidden layer만 사용하여도 존재하는 모든 연속함수를 근사할 수 있다. that a feed-forward network with a single hidden layer containing a finite number of neurons can approximate continuous functions on compact subsets of R 위 그림과 같이 연속함수들은 수많은 아래와 같은 함수들을 사용하면 근사가 가능하다. ..
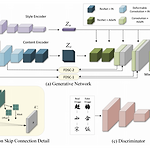
Abstract 현존 methods는 대부분 supervised learning, 매우 많은 paired data가 필요 image-to-image translation은 style을 텍스쳐와 색깔로 정의 냉림 Feature Deformation Skip Connection (FDSC)를 제시 predict pairs of displacement map employs the predicted maps to apply deformable convolution to the low-level feature maps from content encoder style-invariant feature 표현을 학습하기 위해 content encoder에 3개의 deformable convolutional layers를..
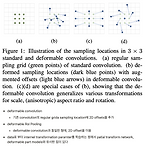
(구조 이해를 위한 짧은 요약) Abstract CNN은 fixed geometric structure의 한계로 geometric transformation에 한계가 있다 CNN의 transformation capacity를 향상하기 위해 2개의 모듈을 제시 deformable convolution deformable RoI pooling spatial sampling location을 offset을 사용하여 변경, offset은 추가적인 supervised-learning 없이 target task를 수행하며 학습된다. 1. Introduction visual recognition task는 geometric variation, model geometric transformation을 핸들링할 수 있어..
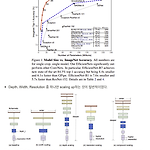
Abstract CNN모델에서 depth, width, resolution 측면에서 세심한 밸런싱을 통해 더 좋은 성능을 이끌어낼 수 있다. 간단하며 효과적인 compound coefficient을 통해 depth, width, resolution의 dimension을 uniform 하게 scaling 하는 방법을 제시한다. EfficientNets은 기존 ConvNets의 성능을 뛰어넘었으며 EfficientNet-B7은 ImageNet에서 84.3% top-1 acc로 SOTA를 달성하였다. 기존 최고 성능 모델과 비교하였을 때 inference에서 8.4x smaller, 6.1x faster Transfer learning에도 좋은 성능을 보였다. (CIFAR-100, Flowers SOTA) 1..
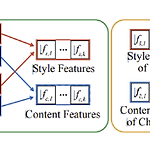
ABSTRACT few-shot generation(FFG)는 2가지 조건을 만족하여야 한다. target char의 global structure 보존 diverse local reference style의 표현 explicit 하게 component label을 주지 않고 MX-Font는 multiple style features를 추출한다. multiple expert들이 different local concepts을 표현한다. 각 expert들이 different local style에 특화될 수 있도록 weak supervision방식으로 component label을 이용한다. 각 expert들에게 component assign problem을 graph matching problem으로 정의..
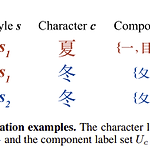
Abstract 기존 Few-shot font generation 모델은 style과 content의 disentanglement을 하고자 하였지만 이와 같은 접근법은 다양한 "local styles"을 표현하는데 한계가 있다 이는 복잡한 letter system(ex. Chinese)에 적합하지 않다 localized styles = component-wise style representations을 학습하는 방식으로 font generation하는 모델을 제안 reference glyph의 수를 줄이기위해 component-wise style을 component factor와 style factor의 product으로 정의한다. strong locality supervision (component의 ..
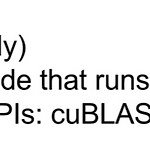
복습이므로 전체적인 내용 리뷰가 아닌 그룹 스터디 중 토론 나눴던 주제 중심으로 정리. 이해하였던 내용을 떠올릴 수 있을 정도로만 정리 Lecture 8 Lecture 9 LeNet-5 conv - pool - conv - pool - fc -fc 형식의 모델 1998년 yann lecun AlexNet ILSVRC에서 작년에 비해 엄청난 성능 향상으로 winner CNN을 활용하는 method가 이후 활발하게 연구 ZFNet AlexNet과 매우 유사한 구조, kernel, filter, stride 등 hyperparameter를 조정 VGGNet 11x11 kernel을 사용하지 않고 3x3 kernel로만 깊게 layer를 구성 3x3 kernel을 깊게 쌓아 large receptive fiel..
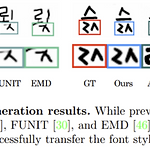
ABSTRACT 글자가 많은 언어에 대해 새 폰트를 만드는 것은 매우 노동집약적이고 시간이 많이 든다. 현존하는 폰트 생성 모델은 많은 reference image를 참고하지 못하면 디테일한 스타일을 살리지 못한다. 조합형 글자에 대해 적은 수의 샘플을 보고 하이퀄리티의 글자를 생성해내는 DM-Font를 제시하겠다 better quality, faithful stylization compared to SOTA font generation methods Introduction GAN base image-to-image translation 방식으로 폰트를 생성하는데 전의 method는 775개의 samples이 필요했다 기존 방식은 pre-trained된 모델에 새로운 reference image를 사용하..
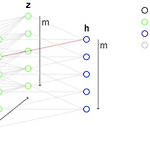
전체적인 내용 리뷰가 아닌 그룹 스터디 중 토론 나눴던 주제 중심으로 정리. Lecture 5 ~ 7 Sigmoid의 문제점 Saturated neuron kills gridients Not zero centered exp() is expensive 만약 input으로 모두 양수가 주어지면 w의 gradient는 모두 양수거나 모두 음수가 된다. w1, w2가 x, y축을 이루는 사분면을 생각해보면 만약 4사분면에 최적의 W가 존재할 경우 w1, w2의 grad는 각각 양수, 음수로 주어져야 빠른 수렴이 가능하다. 하지만 위 상황에서 이는 불가능하여 oscillation 형태로 수렴이 느려진다. Tanh zero centered saturated kill Relu no saturated kill comp..
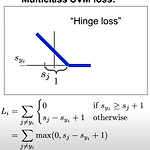
그룹 스터디로 복습 중, 간단하게 잊어버렸던 내용만 정리. Hinge loss 식은 위와 같다. true label에 대한 logits이 각 label에 대한 logits보다 1이상 클 경우 0의 loss를 가진다. +1은 arbitrary choice로 W의 scale에 따라 달라질 수 있는 값. 전체 모델에는 큰 영향이 없다. 크게 엇나가지 않은 값에 대해 loss를 주지 않아 기존에 잘 학습하고 있던 class의 예측값에 대한 변동에 robust하다. Q. W가 매우 작아 s값이 거의 0에 수렴하면 loss의 값은? -log(num of classes - 1) Q. true label을 굳이 빼고 식이 구성되는 이유? 자신 클래스가 포함되면 loss에 +1이 포함됨. zero로 minimun los..
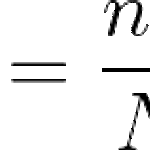
생성 모델링 생성모델은 deterministic이 아닌 probabilistic 모델로 학습 데이터를 나타내는 분포를 최대한 학습하여 그 분포내에서 샘플링하여 output으로 하는 모델. 판별모델링은 x가 주어졌을 때 레이블 y의 확률인 P(y|x)를 추정 생성모델링은 x의 관측확률 p(x)를 추정한다. label이 있다면 p(x|y)를 추정. 생성모델에 대한 연구는 앞으로 인공지능을 이해하는데 매우 큰 도움이 될 것. supervised 판별 모델은 데이터가 생성된 방식에 집중하고 있지 않다. 강화학습 분야에서 강점을 가진다. 사람은 코끼리 이미지를 상상할 수 있음. 지금까지 봐온 코끼리를 정확히 그대로 상상하는것이 아닌 코끼리의 특성을 토대로 분포내에서 상상. 생성 모델이 만족해야하는 조건 (절대적이..
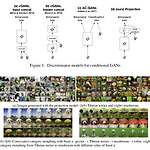
ABSTRACT conditional information을 projection을 기반으로 GAN의 discriminator에게 전달하는 이론을 제시한다. 기존 cGAN은 concat (embedding)형식으로 conditional information을 사용하고있다. Introduction 이안 굿펠로우의 GAN은 이미지 생성분야에서 SOTA 알고리즘이다. GAN의 Discriminator는 생성된 분포인 p_g(x)와 true target 분포인 q(x)의 divergence를 측정한다. 학습하면서 Discriminator (이하 D)가 Generator(이하 G)에게 더 정밀한 측정값을 보내주고 G는 이를 학습하여 target 분포에 가까운 이미지를 생성하는 방향으로 학습된다. cGAN은 clas..
Game Client Programmer (Unreal Engine) & AI Engineer (21.07 ~ 23.08)VoyagerX Deep Learning Engineer Team Ownglyph, Generative model Research & Serving 24.08.22 졸업, 언리얼엔진 클라이언트 프로그래머 구직중 Email: jeong.yjune@gmail.com
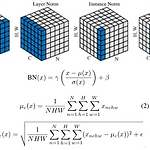
Abstract 기존 neural style transfer의 경우 느린 iterative optimization process를 거친다. 리얼타임으로 동작하기에 너무 느린 속도 Adaptive instance normalization (AdaIN) layer는 content feature를 style feature의 분포와 맞춰준다. x는 content feature, y는 style feature를 의미한다. Introduction 주어진 이미지 x를 weight로 취급하여 gradient descent를 통해 반복적으로 업데이트하는 기존의 neural style transfer는 content, style 퀄리티가 뛰어나나 시간이 오래걸린다는 문제가 있으며 feed-forward method는 하나..
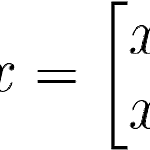
선형대수학에서 Eigenvector는 선형 변환이 일어나도 방향이 변하지 않는 벡터를 말한다. (A - lambda)x = 0에서 ( A - lambda )가 역행렬을 가질 경우 x는 trivial solution인 영벡터가 된다. x가 영벡터를 제외한 값을 가지려면 A- lambda는 역행렬을 가지지 않아야하고 따라서 det( A - lambda ) = 0 구한 Eigenvalue를 (A- lambda)x = 0 식에 대입하여 단위벡터인 Eigenvector를 구할 수 잇다. x1 = 1, x2 = 1 and x1 = -1, x2 = 1 Eigenvector (1, 1) , (-1, 1)은 A에 의한 선형 변환에서 방향이 바뀌지 않고 크기만 바뀌는 벡터다. Eigenvector를 이용하여 Eigen d..
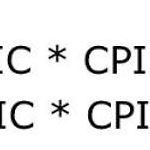
CPU의 성능 평가 CPU Times: Task 완료까지 소요시간 CPU Clock Cycles: Task를 완료하는 데까지 필요한 Clock의 수 Clock Rate: 1초에 몇 번의 Clock을 도는지 (초당 사이클) = 클럭 속도 Clock Cycle Time (cct, Clock period): 하나의 Clock 당 소요시간 (1->0->1) Instruction Count: Instruction의 개수 CPI: Cycles Per Instruction, 하나의 Instruction이 필요로 하는 Clock Cycle의 개수 Formula [ Task 완료까지 소요시간 = 필요한 Clock의 수 x 1개의 Clock당 소요시간] CPU Times = CPU Clock Cycles x Clock C..